Most medium to large businesses are sitting on vast amounts of customer feedback, but they’re unable to make sense of it all.
But that doesn't have to be the case. In 2024, analyzing and interpreting feedback can be simple, fast, and accurate—without any human involvement.
The answer, of course, is customer sentiment analysis. This modern technology has evolved so far that it can deliver clear, actionable insights regardless of how nuanced and complex your customer feedback.
In this article, we’ll take a look at the applications of sentiment analysis for customer insights and how businesses can benefit from it.
Expect to read:
- What is customer sentiment analysis exactly?
- Two examples of sentiment analysis in action.
- How customer sentiment analysis works (with step-by-step and template to run your own manual analysis).
Let's dive in.
What is Customer Sentiment Analysis? Meaning.
Customer sentiment analysis is a data processing technique that evaluates and interprets customers' opinions, emotions, and attitudes from their written or spoken feedback.
This analysis transforms subjective “customer sentiment” into actionable insights, allowing businesses to understand and respond to customer perceptions effectively.
Types of Customer Sentiment Analysis
Most customer sentiment analysis tools categorizes feedback as positive, negative, or neutral. However, there are more advanced types that identify emotions, urgency, and other intentions from customer text data.
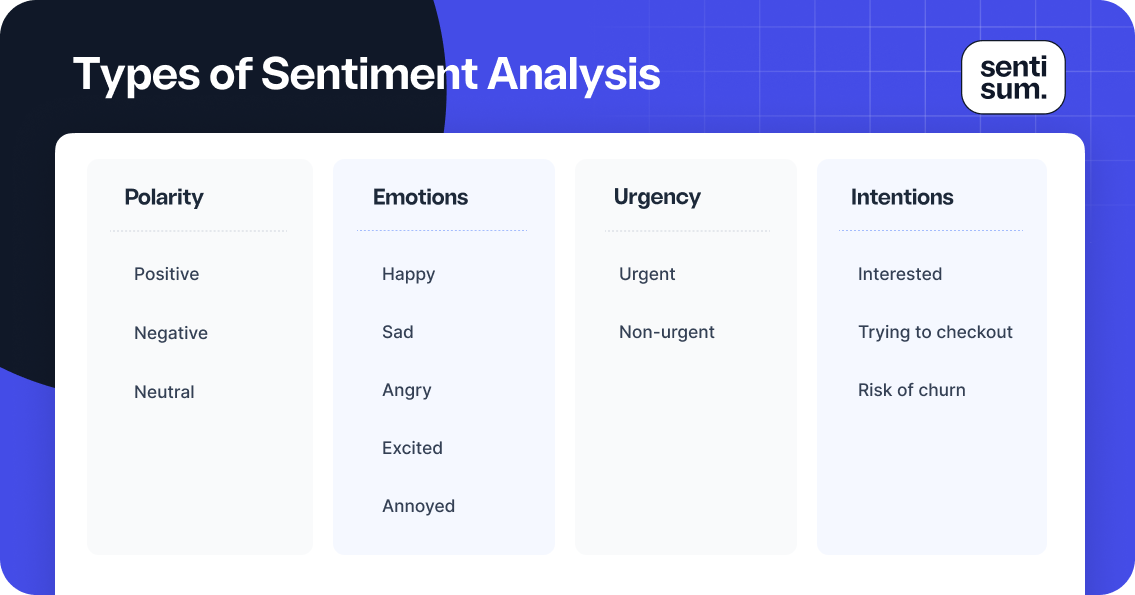
The most important type of customer sentiment analysis for you to be aware of is "aspect-based sentiment analysis":
"Aspect-based sentiment analysis is a more granular approach to understanding customer sentiment. Instead of assessing an entire piece of text as positive, negative, or neutral, it breaks down the text into smaller units called aspects (aka topics and subtopics) related to specific features of a product or service."
For each aspect, it evaluates the sentiment expressed. This method allows businesses to pinpoint exactly what customers like or dislike, providing more detailed insights into customer preferences and areas for improvement.
Let's see some examples of that in action.
Examples of Customer Sentiment Analysis In Action
The goal of a customer sentiment analysis is to understand large volumes of natural language data (e.g. support chats, surveys, or customer review feedback). The insights help companies:
- Understand consumer needs better
- Improve operations, products or services accordingly
- Enhance customer satisfaction and experience
Let’s look at that in action. Starting with sentiment analysis for customer support teams:
1. Example #1: A customer service sentiment analysis
Companies often have thousands (if not hundreds of thousands) of customer support interactions with customers every day.
At that scale, categorizing and analyzing conversations becomes impossible for a single person to do by hand—and as such, nobody has full visibility into the issues customers are facing with your brand.
So, how can sentiment analysis help? With it integrated into your contact center, you can leverage the magic of “aspect-based sentiment analysis” to extract topics and their sentiment from every conversation.
For example, in the image below, our sentiment analysis AI is integrated directly into the Zendesk of a video game company.
All their customer conversation are now categorized with topics and subtopics ("aspects"), and each of those topics is analzed to understand its sentiment.
The result?
The gaming company now understands issues causing their customers to reach out (and the impact of them).

They now know that Game Froze has driven 46,000 customers to contact customer service and only 2% of those were labeled positive.
This data can be used to:
- Encourage other teams to act with more urgency.
- Identify projects to prioritize.
- Auto-route tickets causing particular negative sentiments to an “urgent” team.
Read our full guide to customer service sentiment analysis here.
2. Example #2: A customer review sentiment analysis
Another of our clients, a large international airport, leverages customer sentiment analysis to interpret their customer reviews.
For example, out of the 100s of reviews left by visitors to the airport in the past month, the AI tells us that cleanliness was a hot topic—and that 86% of the mentions were positive.
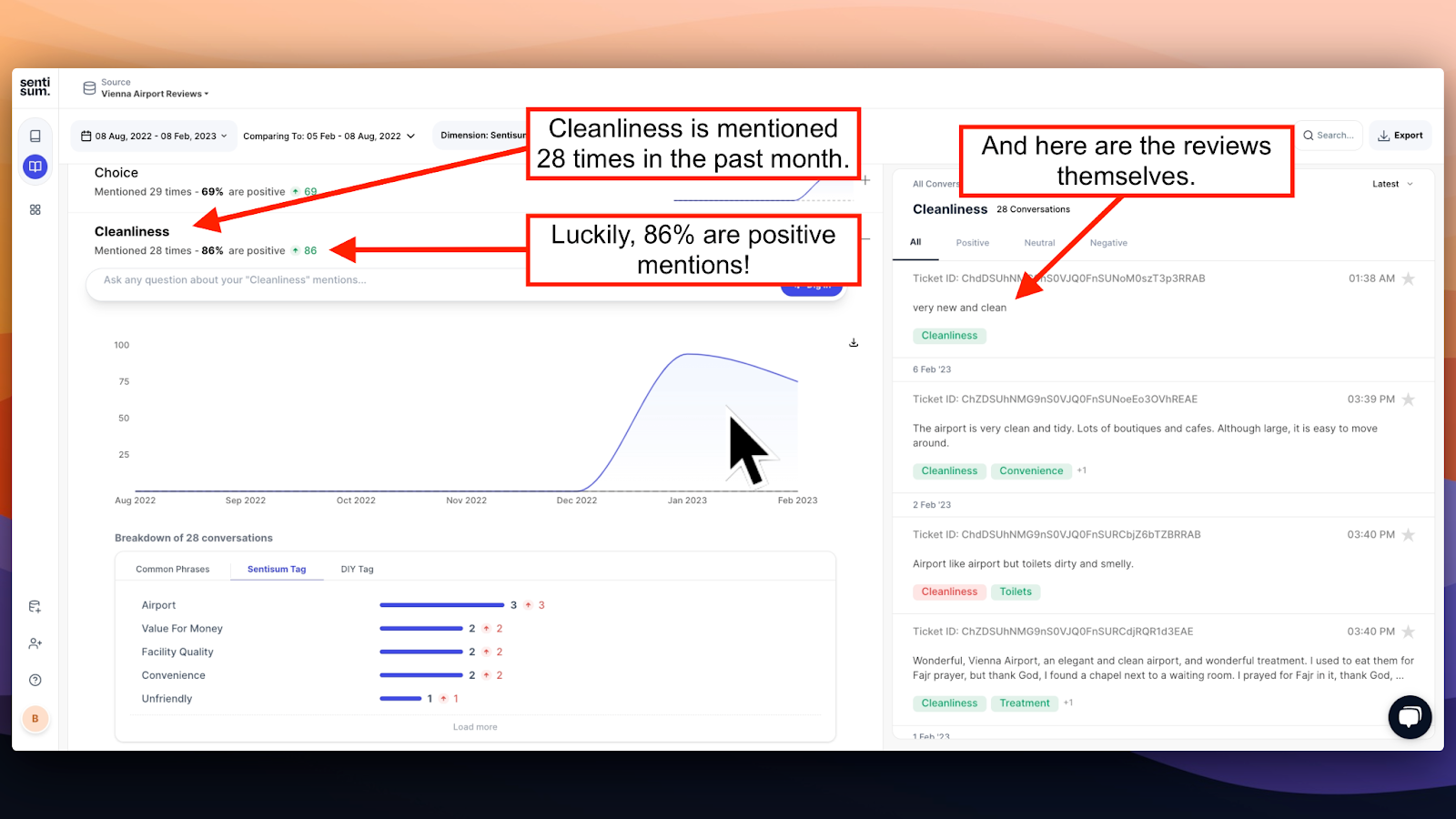
We could interpret this as “cleanliness” is such an important topic that it causes people to leave reviews.
How to Analyse Customer Sentiment Manually (Step-By-Step)
This guide to manual sentiment analysis is meant to illustrate the basic machinery behind sentiment analysis.
If you have more than a handful of customer data to analyze, this will be time intensive. But take a small sample to get familiar with the process.
Not interested in doing this manually? Check out our guide to the best customer sentiment analysis tools here.
Without further ado, here is our guide to manual sentiment analysis:
Manually Analysing Customer Sentiment in Excel
Our guide breaks down into 5 easy-to-follow steps:
- Download the template
- Choose feedback channels
- Collect feedback
- Tag feedback by sentiment
- Report results: present analysis and drive insights
Step 1: Download the Customer Sentiment Analysis Template
Before we get into our rundown of the best sentiment analysis tools out there, we’ve provided a link to the template we used for the above section for you to play around with.
Click below 👇 to download the Google Sheet template and mould it to your team's needs.
→ Free Download (No Email Required)
Step 2: Choose Feedback Channel
The 3 most common feedback channels are Support Conversations, Customer Reviews, and NPS and CSAT Surveys.
Here’s some background on each:
A. Support Conversations are an incredibly underutilised feedback channel, which is a shame considering they easily the most useful source of customer feedback.
Support ticket logs (emails, calls, live chats) contain unbiased, qualitative feedback and we've written extensively about why support is the most valuable source of insights.
B. Customer Reviews are a core driver of sales as most customers look there before making a purchase. This makes understanding the reasons behind negative or positive review sentiment important for business growth.
British Airways currently uses our sentiment analytics tool for their customer reviews, check out this case study to help their NPS score take off ✈️
C. Surveys - NPS and CSAT surveys have long been industry standards. However, with questions being asked about potential bias in NPS scores, you’ll need a discerning customer sentiment analysis tool to validate your survey campaigns.
Step 3: Collect Feedback
Next, you’ll want to collect all your raw data in one place. For our purposes here, we’re using Google Sheets, but Excel or other graphing software can work just as well. Going forward, we’ll use this simple example template:

As you can see, we qualified feedback with 3 taxonomies - Channel, Positive Topic(s), and Relevant Department.
To come up with these taxonomies you can, and should, mould your taxonomical classification to your specific needs.
This is called ‘taxonomical tagging’, meaning creating new labels for your data based on your brand’s specific needs. This process is an art unto itself – it requires careful fine-tuning and attention as its results directly affect the quality of your output.
Both the nature and the complexity of the data you gather is up to you. Think carefully about how you’re going to categorise the data - what’s best for another business might not suit yours. So, ask what groups/ types of customers interest you, then target those channels.
Complexity-wise, if your process can handle starting off with more information, go for it. For instance, you might consider gathering more aspect-based sentiment information - you remember the four types from earlier – Polarity, Urgency, Intent, Emotion – to unlock more advanced results downstream.
The best tagging approaches also invent novel taxonomies to suit their own needs:
Tagging Tip 🎯– One favoured tag by our customers is the “ChurnRisk” tag which helps us to identify in real-time when a customer is experiencing a problem or emotion that will likely lead them to cancel their account or leave for a competition – utilising this tag makes prioritising those customers faster and simpler.
No matter what you do, take the time you need to create your own best practice – remember, ‘Quality in = Quality out’. Looking for more guidance? We got you with our extensive taxonomy best practices guide.
Sounds exhausting? Luckily, automation offers a more accurate, less overwhelming shortcut.
The data we presented above probably seem basic– because it is. Machine learning offers an easier and more advanced approach to sentiment classification.
Sentisum’s automated sentiment tool, for example, uses complex automated labelling practices to speed up the process, empowers it with automation (it comes up with potential groupings for you!), and ensures its insights are both granular (deeper and more detailed) and actionable - continue reading about how it accomplishes this.
Step 4: Categorise Feedback by Sentiment
We've pair our classified feedback with a numeric score - focus on the 'Sentiment' pillar on the far right from our previous table:

See those 1’s and 5’s on the far right? We’ve recorded the negative and positive sentiments detected, but also paired them with a sentiment score on a 1-5 scale. Here’s how we measured those numbers:

More complicated for a reason 😏: Customer experience experts will notice the similarity to NPS scoring. Our ratings here are determined using a similar scale but are uniquely useful in this case as they complicate our data in a useful manner.
Here’s how: These ratings allow us to determine the best approach for each variety of sentiment. A ‘1’ rating, meaning Happy feedback, should be noted for its success and applied to future business practices. A ‘5’ rating, meaning ‘Angry’ feedback, on the other hand, indicates an issue that requires an immediate fix.
By doing this, we’ve taken our categorised feedback – the feedback we broke into groups by sentiment – and further understood it, breaking it into micro-groups, by type of sentiment.
At this point, you’ve collected our data, classified it, and ranked it by sentiment. You’re ready, at long last, react to use complex customer sentiment analysis to distil your data for results 😎
Step 5: Report Results: Present Analysis and Drive Insights
If you use the right taxonomies to rediscover your data, then rank their sentiment, you’re ready to take the final step and uncover new, previously-unknown nuggets of knowledge - aka insights. A good rule of thumb for reporting sentiment analysis data is that:
Accessible insights are actionable insights 🙌
The final step of the process, ‘reporting’ your insights, means making them understandable to everyone that might need them, from highly technical employees to those without much knowledge in the area. Your results need to be clear and trustworthy.
How can you test for this? We will get into some examples, given that reporting techniques are one of our specialties at SentiSum, we’d be remiss not to set you up with a few in-depth resources first 👀:
For experts looking for deep knowledge, we’ve compiled an ebook on presenting analysis results internally.
Or, for those looking for a quicker fix, here’s our outline of our survey report examples.
Check either for explainers on everything customer sentiment reporting - your teams and your brand will thank you.
On to our example 😤:
4a. Time-Series Report:
Take a look at a 'Time-Series Report' - this report tracks the percentage change of tags over a given period of time - here's a sample report generated by our sentiment analysis tool:
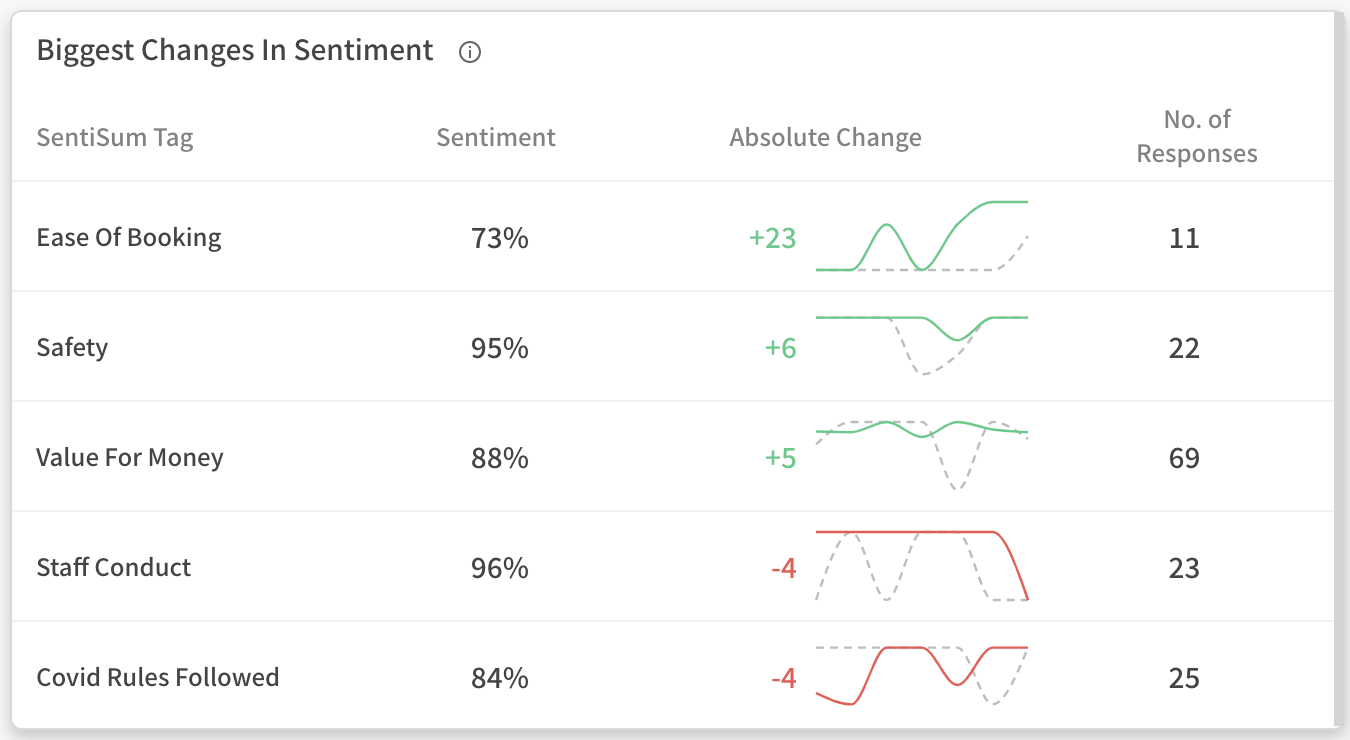
Time-series change is a great place to start because it offers clear data that should spark direct action - or that’s the hope.
If an untrained eye looked at this graph the Absolute Change +/- and the graphs that accompany it jump off the page. It’s clear that Ease of Booking is doing great and that Staff Conduct and Covid Rules Following are drawing complaints.
Using this chart, any level of employee would be able to conclude that the Conduct and Covid Rules Following need improvement and should be able to start putting a plan into action, treating the new pain point uncovered by your analysis 🩹 - a superb example of accessibility transforming insight into actionable insight.
Automated Sentiment Analysis Solutions
If you have more than a handful of monthly customer feedback and support conversations, automation is critical. It makes sentiment analysis coherent and accurate—allowing your company to trust the insights to make better decisions.
We wrote a guide to the top 6 customer sentiment analysis tools here—if you're interested in a thorough review of tools on the market, please take a look there.
In this guide, I'll walk you through our own customer sentiment analysis tool, SentiSum.
SentiSum - Customer Sentiment Analysis for Customer Service Teams
SentiSum is an AI-powered platform that consumes, understands, and gives detailed insights on your customer feedback (any channel—from call centre conversations to surveys).
We’ve built the platform to be simple to use and customizable, so that everyone in your organization can benefit from customer experience insights.
If you’re in a mid-market and enterprise business, the number of chat messages, calls, emails, and sentiment surveys that your support teams receive can add up to hundreds of thousands of interactions a month.
Your customer support/experience team likely doesn't have the capacity to handle this volume of support tickets. Instead, you might try a different strategy to understand what customers are saying:
- You could extrapolate insights from just a sample of sentiment data. But this way, you won’t really have confidence in those insights. Or,
- You could periodically undertake a complete analysis of customer support sentiment. Yet, by the time you complete that, the insights are usually out of date.
SentiSum solves these problems by bringing all your customer support conversations and survey responses into one easy-to-use platform.
This way, you can access deep, timely insights on what customers are saying, to drive improvements you can feel confident about.
Book a demo with us to see the tool in action.
Related Reads: