AI analytics for customer complaints.
SentiSum makes customer complaint analysis accurate, detailed, and lightning fast. Quantify free text feedback, identify trends, and make confident CX decisions.

How it works
Sentisum uses the latest advances in natural language processing to analyze your customer complaints wherever they are—calls, chats, emails, reviews, and survey results included.
It doesn't read and analyze like a human. We get repetitive things wrong and we're slow.
Instead, it combines human-levels of language understanding with the speed and uniformity of AI.
Our platform makes your complaints accessible, so you can understand exactly what's impacting your customers.
Automated customer complaint analysis that gets to the root cause
SentiSum's machine learning-based analytics uncovers granular insight, whatever the scale of complaints you have.
You'll suddenly have access to quantitative topics and their sentiment in real time, in enough detail to uncover what's responsible and work on a fix.
Turn your customer complaints into an answer engine
Say goodbye to manually digging through data or waiting on reports. Get quick, meaningful answers about your customer experience with SentiSum's AI answer engine. Ask any question you want and get an answer based on your real complaints.
Get daily updates on the drivers of customer complaints
Our daily digest email ensures you stay up-to-date with performance, top ticket drivers and the largest rises and falls in topics.
Subscribe anyone to bring company-wide access to support data. You'll see a refocus on customer experience that helps you to reduce ticket volumes.
Why replace your existing tagging system?
Most tagging systems take a lot of manual work or the insights can't be trusted to back up business decisions. Usually tags are inaccurate, inconsistent or generic, so customer support is like a black box.
Before SentiSum
• Tags are broad and require manual digging.
•Tags become outdated so insight is missed
•Tags are based on 'keywords' = inaccurate
•Tags are applied inconsistently by agents
•Reporting is still time-consuming
After SentiSum
• Tags are granular and get to the heart of the issue.
• Tag taxonomies are continuously up-to-date
• Tagging is machine-learning based = accurate
• Tags are applied consistently to 100% of your tickets
• Reporting is made simple with automation
• Tags can be trusted to guide triggers, automations and company-wide improvements
All your channels under one roof
SentiSum is a single source of truth. In one simple-to-use dashboard, you'll understand the topic and sentiment of every customer conversation, survey and review.
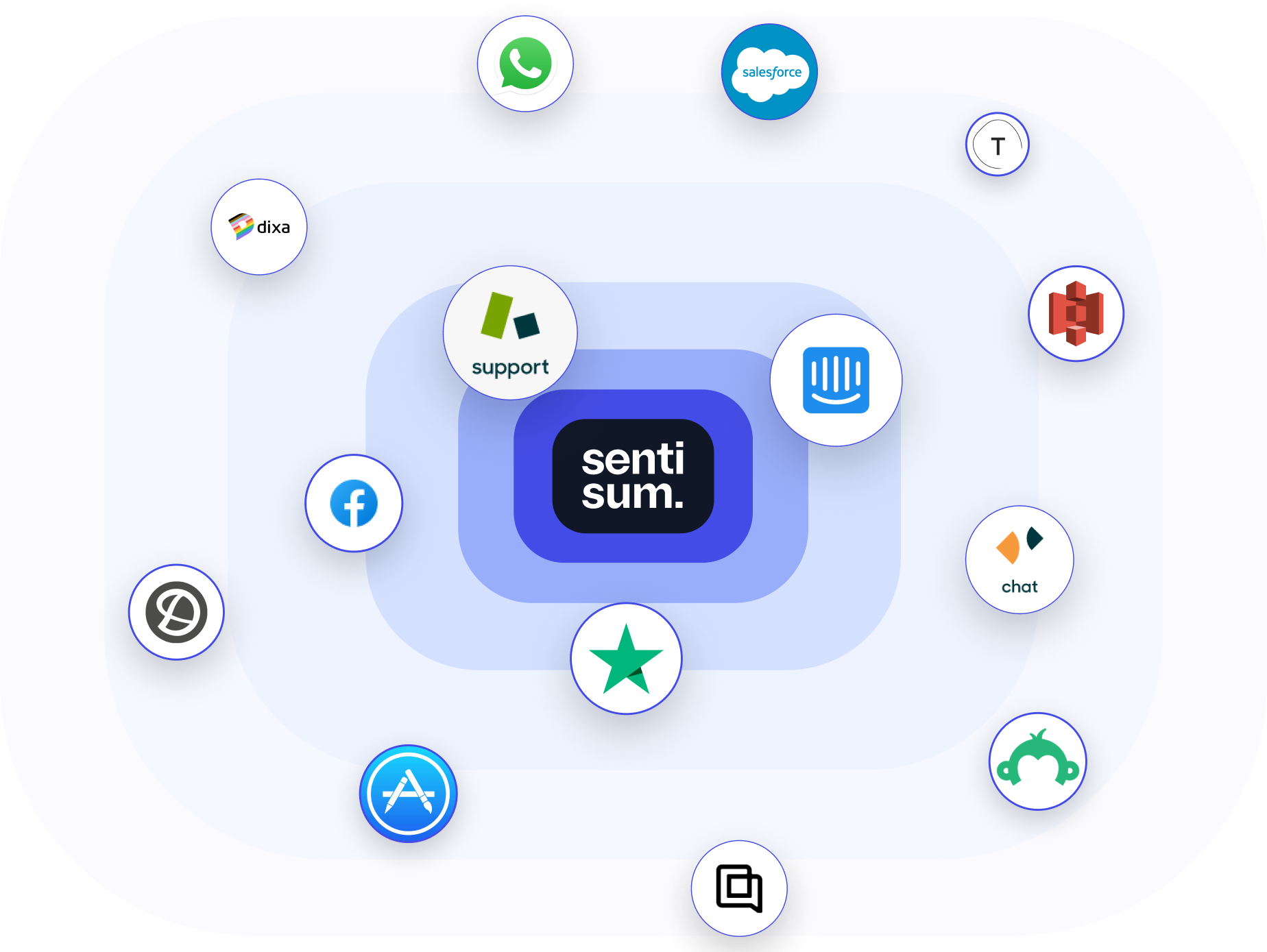
Try SentiSum today
Turn every customer feedback into clear, easy-to-use insights.
Customer Complaints Analysis FAQs
What is customer complaint analysis?
Customer complaints analysis is a process used by businesses to evaluate and understand the feedback, especially negative or critical, provided by their customers.
This analysis helps companies identify common issues, potential areas for improvement, and opportunities to enhance customer satisfaction.
These are the key aspects of a customer complaints analysis:
- Data Collection: The first step involves gathering customer complaints from various sources such as direct feedback, emails, social media, reviews, and customer support interactions.
- Categorization: Complaints are categorized based on common themes or issues, such as product defects, service delays, or billing problems. This helps in identifying the frequency and severity of each type of complaint.
- Root Cause Analysis: By digging deeper into each category, companies can identify the underlying causes of complaints. This might involve analyzing processes, employee actions, or external factors affecting customer satisfaction.
- Trend Analysis: Over time, analyzing trends helps in understanding whether the number of complaints in certain categories is increasing or decreasing. This can be crucial for detecting emerging problems before they become more widespread.
- Resolution and Feedback: Effective complaint analysis includes resolving the issues identified and communicating back to the customers about the steps taken. This can help in rebuilding trust and improving customer relationships.
- Continuous Improvement: Insights gained from the complaints are used to make informed decisions about product improvements, service enhancements, or changes in business processes. This ongoing process contributes to continuous improvement in the quality of customer service and product offerings.
As you can imagine, this is a valuable tool for any business aiming to improve its customer experience, reduce churn, and enhance overall operational efficiency.
Why is customer complaints analysis important?
Customer support is frequently described as a 'black box'. There's endless amounts of data hidden away in those customer conversation and complaints but it's largely inaccessible. Uncovering that data is critical. And reporting it companywide creates a competitive advantage.
When you create access to complaints, by quantifying large volume of qualitative data, you can help your team to start tackling bad CX, customer disatisfaction and drivers of churn in a heartbeat.
How does AI text analysis categorize customer complaints?
AI text analysis categorizes customer complaints using several advanced techniques that automate and enhance the process of understanding and organizing customer feedback.
Here’s how it typically works:
- Feature Extraction: AI uses techniques to understand and quantify the text. We use machine learning, so our AI doesn't rely on keywords of phrases, but truly understands the meaning of language—even if mispelled or confused.
- Sentiment Analysis: The AI assesses the sentiment of the text, determining whether it's positive, negative, or neutral.
- Classification: Based on the features and sentiment, the AI categorizes each complaint into granular categories and subcategories such as "late delivery" and "blue packaging damaged". The categories are continuously refined based on common issues that arise.
- Machine Learning Models: AI employs machine learning algorithms to learn from the data and improve its accuracy in categorizing new complaints over time.
This streamlined approach allows businesses to efficiently sort and address customer complaints based on their content and sentiment.
What are some examples of the benefits of complaint analysis?
Complaint analysis offers a variety of benefits to your business, aiding in both strategic decision-making and operational improvements.
Here are some key examples of these benefits:
- Improved Customer Satisfaction: By understanding and addressing the issues that lead to complaints, you can improve your experience at every touchpoint, which directly boosts customer satisfaction and loyalty.
- Product and Service Improvement: Complaints provide direct feedback on what might be wrong with a product or service. Analyzing these insights allows companies to make targeted improvements, thereby reducing future complaints and enhancing the overall quality of offerings.
- Operational Efficiency: Identifying recurring complaints can help pinpoint inefficiencies or breakdowns in business processes. Resolving these issues can lead to smoother operations and reduced costs.
- Risk Management: Effective complaint analysis can help in early identification of potential risks, such as defects in products or gaps in service that could lead to larger issues if not addressed promptly.
- Customer Retention: By responding to and resolving complaints effectively, you can retain dissatisfied customers who might otherwise switch to competitors. This retention is often more cost-effective than acquiring new customers.
- Brand Reputation: Proactively managing and resolving complaints can improve a company’s reputation. A reputation for valuing customer feedback and quickly addressing issues can distinguish a company from its competitors.
- Regulatory Compliance: For certain industries, monitoring and reporting on complaints is a regulatory requirement. Effective complaint analysis ensures compliance and can help avoid legal or regulatory penalties.
How do we get started?
Sentisum is quick to setup and get started. Reach out to our team or a product tour here, we'll show you how it works and walk you through the next steps. Our customer success team will do all the heavy lifting to getting started and will be with you every step of the way.