Customer reviews tell you so much about:
- Your best customers and what they like about you
- The biggest customer complaints
- Product improvement areas
But if you have thousands of reviews, how do you make sense of them all?
You could manually read and label each, but it’s incredibly time-consuming. So, you end up analyzing just a sample, which doesn’t fully represent your customers’ feelings.
A more accurate and consistent approach is to use a sentiment analysis software, like SentiSum.
These tools use AI to analyze and tag all your reviews in minutes, and tell you the key issues and overall sentiment—whether it’s negative, positive, or neutral.
In this article, we’ll expand upon these two main methods to conduct sentiment analysis of reviews:
- The Manual Method (suitable for <100 reviews)
- The Software Method (Suitable for >100 reviews and Customer Experience leaders who want a continuous, uniform approach)
What is Sentiment Analysis for Customer Reviews?
Sentiment analysis for reviews is the process of breaking down and classifying customer reviews into positive, neutral, or negative.
You can calculate the overall sentiment from your reviews using this formula:
Sentiment (%) = (Number of Positive Mentions / Number of Overall Mentions) * 100
Sentiment analysis is the holy grail of insights for Customer Support leaders for three main reasons:
- Sentiment analysis tells you what customers love about you—whether it’s the stellar customer service or the product’s ease of use. You can then highlight these testimonials on your website or other marketing materials.
- Sentiment analysis tells you the main friction areas in your customer’s journey. This way, you know exactly what your next improvement project should be.
- Sentiment analysis tells you which product features you should improve or create. This gives you the data (and the confidence) to answer the product team’s ad-hoc questions and lead development projects.
So, how do you go about getting these insights?
📖 Related read: How Can Sentiment Analysis Improve the Customer Experience? [8 Use Cases]
How to Analyze Sentiment From Reviews [2 Ways]
There are two main methods to analyze sentiment from reviews:
- Manual data analysis. For small datasets of less than 100 reviews, you can look at each one individually and search for patterns and trends. We don’t recommend this for companies with large volumes of reviews, however.
- AI-based sentiment analysis software. If you have over 100 reviews per month, and you want a consistent and uniform approach, you can use an AI-powered solution like SentiSum.
Let’s look at each in detail.
Method #1 - Manual Data Analysis - Suitable for <100 Reviews
In manual data analysis, a human (either an agent or the Customer Support Leader) reads through each review and labels it with a topic and sentiment.
For instance, if you have a review like:
"I've been using [Service Name] for a few months and love the variety and flavors of the meals, which are a great fit for my busy schedule. However, the amount of plastic packaging is excessive. It would be perfect if they could switch to more eco-friendly options."
You’d tag it as:
- Topic: Plastic Packaging, Sentiment: Negative
- Topic: Meal Variety, Sentiment: Positive
Here's how you can manually analyze the sentiment behind reviews:
- Collect all your reviews in one place.
- Read through each review thoroughly. Determine the overall sentiment (positive, negative, or neutral) based on the tone and language used in the review.
- Categorize the review into a specific topic based on the main subjects discussed, such as delivery, quality, or customer service.
- Input the sentiment and topic into a tracking system or a spreadsheet.
- Repeat no. 2 to 4 for all reviews.
- Analyze the compiled data to identify common themes or recurring issues to inform business decisions.
This is fine if you have only a handful of reviews, but you can imagine how this can quickly snowball into a huge task if you have thousands.
Also, there are three main inherent problems with manual labeling.
- Because manual labeling is so time-consuming, you can only analyze a sample of reviews. This sample data might be anecdotal, non-representative or missing a glaring issue.
- Manual labeling is biased by the analyst doing the tagging.
For example, if a review says, “My meal kit arrived late but I loved the meal,” one of your agents might classify it as negative (“shipment arrived late”) while another might say it’s positive (“loved the meal”).
Also, if one employee leaves and another takes over, it's likely that they understand reviews differently, making topics and sentiment subjective.
- The length of time taken for the manual work detracts from other priorities. This means in some months, you might not be able to do manual analysis, resulting in an incomplete picture of brand drivers over time.
That’s why we recommend most mid to large businesses use sentiment analysis software. This helps create a more consistent, automated approach to categorizing and understanding customer reviews.
Method #2 - Using AI-Based Sentiment Analysis Software - Suitable for a Consistent Analysis of Reviews at Scale
AI-based sentiment analysis software uses Natural Language Processing to read through reviews (much like a human would) and labels each with a predetermined tagging taxonomy. This includes:
- Topic
- Sentiment
- Intent
- Priority
This method is ideal if you have large volumes of reviews across different platforms. You’ll get a fast, yet thorough and precise picture of customer sentiment.
There are different types of sentiment analysis software, but we recommend you choose one that uses machine-learning based NLP.
Machine-learning based NLP understands speech and text in a similar way to humans. After digesting a dataset being trained in it, it uses statistical inference to carry that knowledge into new environments it’s never seen before.
For example, it can identify and infer the meaning of misspellings, omitted words, and new words like slang by itself.
Thanks to the ML, it learns the patterns between phrases and sentences and constantly optimizes itself to improve accuracy over time.
In the image below, you can see how powerful machine-learning NLP is compared to keyword extraction (what platforms like Zendesk typically use) and rule-based NLP (what other software providers use).
Learn more about different types of NLPs here.

This is why we built our sentiment analysis tool, SentiSum, using machine-learning based NLP.
In a nutshell, here’s how it works:
- SentiSum scans and analyzes thousands of reviews, from multiple channels, in real-time.
- It uses advanced machine learning-based Natural Language Processing (NLP) to categorize reviews as positive or negative and understand the nuance and context behind each.
- It helps you spot trends, quantify issues, and get actionable insights to make better business decisions.
Let’s look at how SentiSum gives you more accurate and consistent insights compared to the manual process.
📖 Related read: 6 Best Customer Sentiment Analysis Tools in 2024
How a Customer Review Sentiment Analysis Works in SentiSum
SentiSum takes the overwhelm out of sentiment analysis for customer reviews and turns it into a streamlined, insightful process.
Let’s dive into how it does this exactly, using hypothetical reviews from Trustpilot about a pet food brand as an example.
1. Compile and Analyze Reviews and Customer Feedback from All Channels
Imagine having to gather all of your customers’ pet food product reviews from across different websites and then trying to make sense of them.
Even if you are tech-savvy enough to know how to “scrape” this information from every potential source (hint: the average person isn’t), the process is rather time-consuming.
With SentiSum, you can pull in reviews and customer feedback from all other channels, such as emails, chats, phone calls, surveys, and more, onto one dashboard.
All you need to do is choose your channel source and authenticate the connection:

You can also add custom integrations with any channel of your choice using APIs.
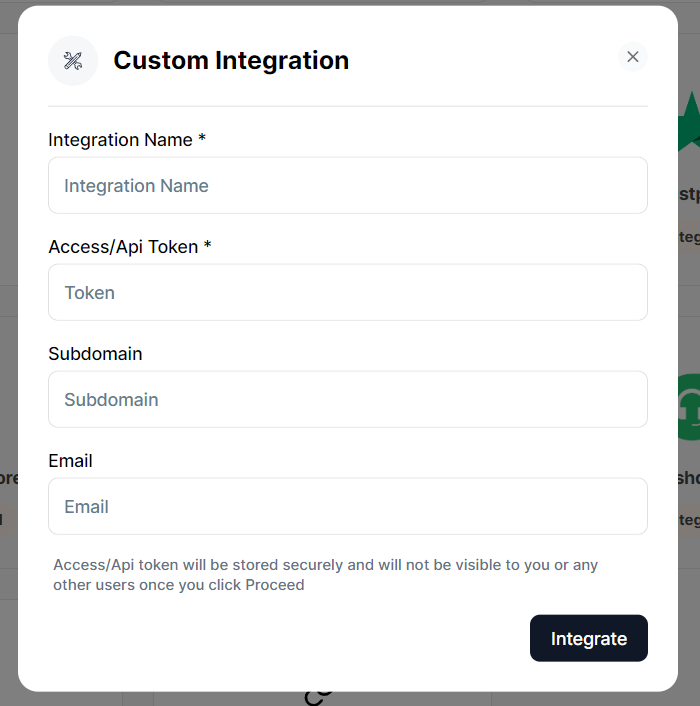
2. Get Accurate, Granular Insights into Key Sentiment, Topics, and Trends
When you manually comb through reviews, your feelings and opinions can influence how you perceive what customers are saying.
SentiSum uses machine-learning based Natural Language Processing (NLP) model which reads every review automatically to determine:
- how customers feel
- why they contacted you
- and how urgent their issue is
This provides more accurate and detailed insights than manual analysis, as AI removes bias and subjectivity.
We asked Kirsty Pinner, Chief Product Officer at SentiSum, why AI is such a powerful tool in sentiment analysis. Here’s Kirsty’s answer:
“AI can cut through the subjectivity of human opinion, and no matter how something is said, it can report on the customer issue in a simple way,” said Kirsty Pinner, Chief Product Officer at SentiSum.
“The latest developments in AI analytics can handle complexity extremely well,” she added. “No other method gives a representation of customer conversations this accurately. Manual tagging is too subjective and trying keyword analysis is too blunt a tool.”
Then, all these key topics and issues are populated on an easy-to-use and easy-to-understand dashboard like so:

3. Dig into Each Topic to Prioritize Fixes
The dashboard shows you all the key issues and top negative sentiment drivers. But how do you know which of these you should fix first?
On SentiSum, you can immediately see increases and decreases in sentiment for a particular topic.
You want to prioritize fixing topics that are decreasing in positive sentiment.
For instance, you may find that reviews related to your pet food’s pricing have gone down in positivity.
Your SentiSum data can give you more insights as to why this is happening.

The tool’s ability to track the volume and sentiment of topics over time means you're not guessing what to fix first—you're making informed decisions based on real data.
It points you directly to what's bothering your customers the most so you can prioritize improvements that cater to customer satisfaction.
4. Stay on Top of All Your Reviews
For businesses that get hundreds or thousands of reviews from consumers, manually reading through each one isn’t viable.
This means you’re potentially missing out on crucial feedback.
SentiSum ensures no review ever slips through the cracks by sorting each review into clear Topics and Subtopics.
You can easily sift through all your feedback to find exactly what you’re looking for.
For instance, if you want to further investigate individual reviews that mention pricing, just click on the Price topic in SentiSum’s dashboard to view all relevant reviews.
What if those reviews are in languages you don't speak? No problem.
SentiSum automatically translates them into your preferred language to ensure every piece of feedback is accessible, understandable, and actionable.

5. Save Time with AI Answers About Your Reviews
If you’re trying to manage and improve a product or service, dedicating hours to combing through customer feedback is both impractical and nearly impossible.
With Dig In, SentiSum’s built-in generative AI feature, you essentially have your own ChatGPT to decipher customer feedback.
Ask the AI anything, and it’ll give concise, summarized answers about your customer reviews.

This saves your Customer Support team massive time on making sense of customer reviews.
If you’d like an accurate, fast, and consistent approach to sentiment analysis, try SentiSum. Book a demo below.

5 Ways Your Sentiment Analysis Review Software Can Drive Change & Improvement
The real value of sentiment analysis lies in what happens when you leverage these insights to make business decisions.
Here are five ways you can use the insights from your customer sentiment analysis software to drive positive change for your business.
1. Understand and Improve NPS Scores
Net Promoter Score (NPS) measures how likely a customer is willing to recommend your company.
But looking at NPS scores alone isn’t enough to make useful improvements. Most NPS surveys are incomplete or not even filled out in the first place.
This is why you need another layer of sentiment analysis of reviews. By analyzing what customers are saying publicly online, you can understand what’s driving low (or high) NPS scores.
For instance, British Airways Holidays had 100,000s of reviews. They wanted to understand what was driving positive sentiment so they could double down on what was driving brand advocacy.
Previously, the team was manually labeling each review but this caused more problems than it solved.
Using SentiSum’s machine-learning AI, the BA team could quickly spot trends and issues affecting the NPS score.
Equipped with these insights, the BA team quickly focused on improving areas that drive customer loyalty and address problems before they affect more customers.
This proactive approach not only enhances customer experiences but also boosts their NPS by turning more customers into promoters.
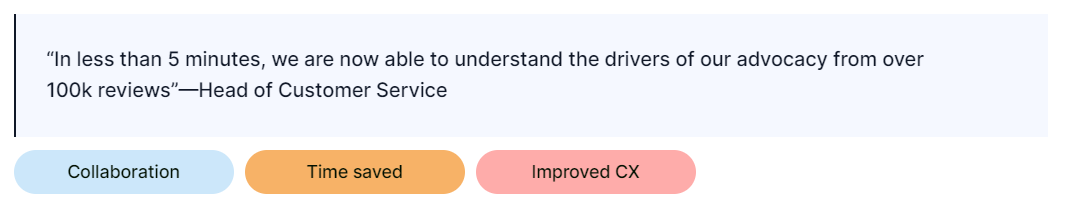
2. Know Exactly Which Product Feature / Service to Improve Upon
The built-in advanced AI features of sentiment analysis tools like SentiSum offer a fast and accurate way to understand which features your customers love and which ones cause frustration.
The detailed and unbiased tags that are automatically generated for every review help pinpoint recurring issues and pain points for your customers.
Take, for example, the experience of Scandinavian Biolabs, a company that leveraged SentiSum to better understand its customers’ feedback.
Before SentiSum, the team was hesitant to make changes.
As CEO Anders Reckendorff noted, "It’s very difficult to convince the rest of the team that something was important when you don’t have the data to back it up."
Data from SentiSum transformed Scandinavian Biolabs’ approach to its strategic and product meetings.
“Insights from SentiSum started becoming the basis for our strategic and product meetings, giving us a sounding board for understanding our customers and their needs outside of customer surveys,” said Reckendorff.
3. Improve Customer Satisfaction and Cultivate Loyalty
Offering recourse to customers who've had a less-than-stellar experience with your product or service can turn a customer’s negative review into a positive customer service story.
With SentiSum, you can quickly identify these negative experiences and focus on outreach to smooth things over.
Conversely, you can also track positive sentiment among customers and shine a spotlight on the “success stories” of happy customers.
You can then engage those customers to become your brand champions, which not only attracts new customers but also reinforces the loyalty of existing ones.
4. Create Agent Training Modules Around Customer Reviews
When your business can quickly identify reviews that mention a negative customer service experience, it becomes easier to understand where your team can make improvements.
SentiSum allows you to pinpoint exactly where your current customer support approach might be lacking based on real customer feedback.
From there, you can use that data to create targeted training modules for their employees.
You can even prepare your agents to handle specific types of concerns SentiSum identifies from customers, such as product bugs.
This type of focused training ensures customer service agents are equipped to handle customer concerns more effectively and improve service quality across the business.
5. Build Evidence-Based Cases to Create Change Across Departments
You probably already have a hunch about the top customer service issues your company faces based on informal feedback from your team of agents.
However, hunches without hard data rarely help to convince other departments to prioritize changes or fixes.
SentiSum provides quantifiable evidence from real customer feedback to support your hunches.
Imagine how much more impactful your request to fix a product feature would be if you could demonstrate that 17% of all negative reviews are related to that specific issue.
Instead of waiting months for a manual data analysis (that will likely be outdated by the time it’s completed), you can use real-time SentiSum data to quickly and effectively create change within your company.
Final Thoughts on Sentiment Analysis for Reviews
- Sentiment analysis transforms overwhelming amounts of customer feedback into actionable insights.
- SentiSum offers a detailed, unbiased understanding of customer sentiment with its AI-powered tools and features.
- Deeply understanding the customer sentiment of your reviews across platforms empowers teams to improve products, services, and customer satisfaction.
- Sentiment analysis software can help drive cross-departmental change with evidence-based data.
