Support conversations are a treasure trove of insights into your customers’ needs, preferences, and frustrations.
But traditionally, they're so hard to analyze because:
- They're qualitative and informal (How do you quantify customer complaints on a phone call?)
- They're high in volume (If you’re a mid-sized company, you probably have hundreds of calls, emails, and chats every day.)
Until now.
Customer conversation analytics tools use AI and natural language processing to capture, analyze, and report on support conversations at scale.
The result? You get granular, actionable insights that actually help you improve your product, customer experience, or teams.
In this article, we’ll explain what it is, how it works, and how it improves customer experience using 6 real-life case studies.
Jump Ahead:
- What is Customer Conversation Analytics, and Why Does it Matter?
- How Does Conversation Analytics Software Work? [4 Parts]
- 6 Real-Life Case Studies of How Conversation Analytics Improves Customer Experience
- 3 Things to Look for in a Conversation Analytics Software
What is Customer Conversation Analytics, and Why Does it Matter?
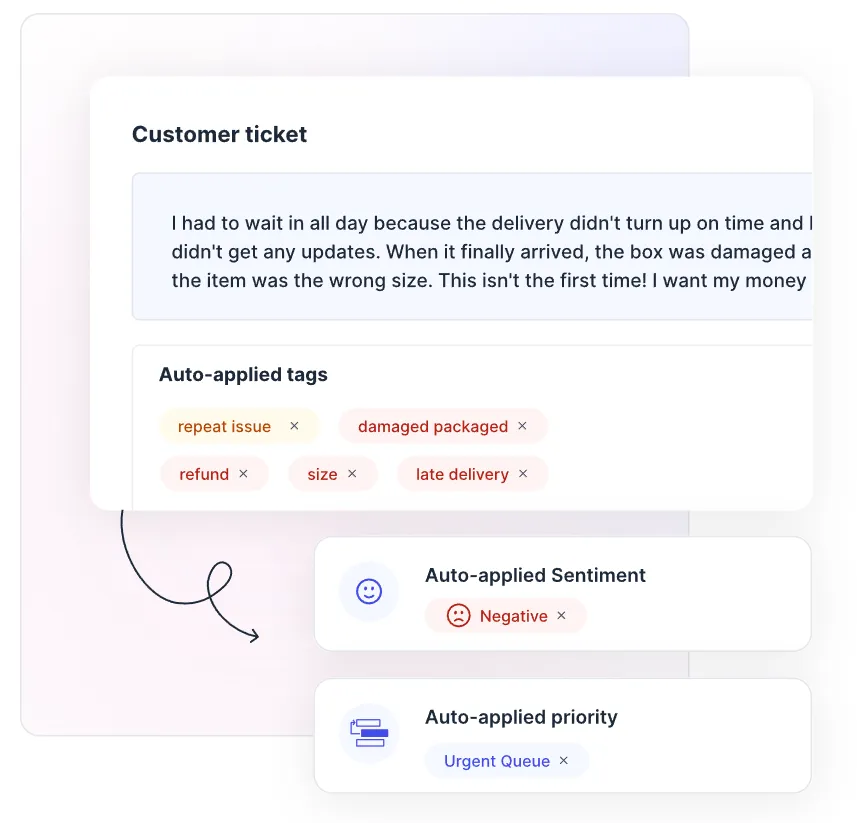
Customer conversation analytics uses AI and natural language processing to analyze your support conversations (calls, emails, chats, and more) at scale. This helps you understand why customers are contacting you and how they feel.
Simply put, NLP is how we can get computers to understand language—speech and text.
Unlike humans, computers are incredibly fast, consistent, and unbiased in their approach.
When a conversation analytics tool processes text in large batches—let's say 100,000 emails—it can be done in an instant, and the results are actionable.
Kirsty Pinner, Chief Product Officer at SentiSum, our conversation analytics tool, says:
“AI can cut through the subjectivity of human opinion, and no matter how something is said, it can report on the customer issue in a simple way. No other method gives a representation of customer conversations this accurately."
Out of all the NLP models, however, machine-learning based NLP produces the most granular and accurate insights.

It relies on more modern ‘statistical inference’ techniques and understands speech and text like a human would.
Once it’s learned to understand human language in a particular environment—say, the legal world—it can infer the meaning of misspellings, omitted words, and new words without a human setting up a new rule.
Machine-learning also constantly optimizes and evolves itself so the accuracy improves over time.
In the image below, you can see how much more accurate and granular machine-learning is, compared to keyword extraction and rule-based NLP.

Let’s look at an example →
Say you’re a retail company and you receive a phone call from a customer who wants a refund.
The customer says their package was delayed without an update and then arrived damaged and in the wrong size.
The machine-learning NLP would transcribe this call, read the text, and label it with a predetermined tagging taxonomy like:
Main Reasons for Contact (or “Topics”): Late Delivery, Refund, Damaged Package
Sentiment: Negative
Priority: Urgent
This means, as a support leader, you’ll be able to:
- Gain comprehensive insights: Analyze all customer conversations—not just a sample—and get a full-picture of what customers really think about your product, service, and brand.
- Build evidence-based cases for change: No more relying on gut-feeling or hunches. Customer conversation analytics turn qualitative conversations into quantitative data that you can use to drive improvement projects that make a difference.
- Improve customer experience: Quickly resolve urgent issues (reducing first-resolution time), lead improvement projects that remove issues at the root, and train agents better to deliver exceptional service and build customer loyalty.
But is it as good as it sounds? Let’s dig a little deeper into how a conversation analytics tool works, and whether it can bring you these actionable insights.
📖 Read more: Speech Analytics for Call Centers: 5 Use Cases & Tools
How Does Conversation Analytics Software Work? [4 Parts]
To make things easier, we’ll use our own conversation analytics tool, SentiSum, to demonstrate how it extracts useful trends and insights from conversational data.
💡For the visual learners, here’s a 40-sec video of how it all works:
Step 1: Integrate with your existing customer conversation channels.
If you have 1000s of conversations, manually gathering them into one place is time-consuming (and often frustrating).
On SentiSum, you can pull data from any channel you’d like in one click. Just authenticate the connection, and your data will automatically flow in for further analysis.

Now your customer support team has a comprehensive view of customer interactions and trend data without switching from one channel to another.
Step 2: Analyze customer conversations at scale using machine learning-based NLP.
SentiSum uses machine learning-based NLP to analyze customer conversations at scale with extremely high accuracy and efficiency.
Unlike traditional rule-based NLP, SentiSum’s model learns from every customer conversation to automatically tag your support tickets as they come in and ensure consistent, detailed insights.

Think of this tagging process as the magical Sorting Hat from Harry Potter.
The Sorting Hat assigns each Hogwarts student to a “house” based on their unique traits, just like SentiSum assigns Topic Tags to conversations based on their content.
Over time, machine-learning learns from user input and becomes better at accurately identifying conversation patterns.
This AI tagging is so much more accurate and consistent than manual tagging, so you always get insights you can rely on for business decisions.
Step 3: Quickly see granular insights on an easy-to-use dashboard.
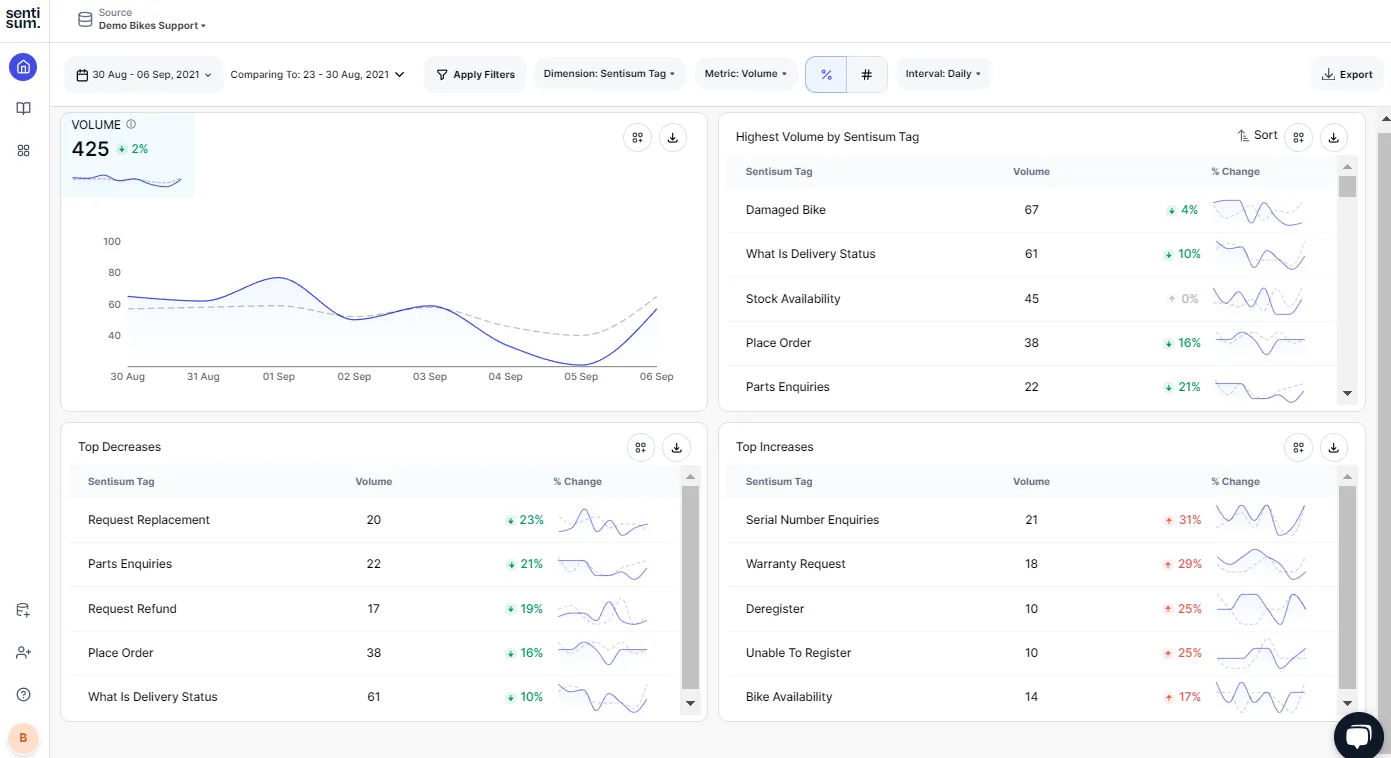
SentiSum's easily accessible dashboard gives you a wealth of granular sentiment insights pulled from your customer conversations.
Here, you and your team will find:
- Top Reasons for Contact so you know the key friction areas for customers.

- Increasing and Decreasing Issues so you can judge whether past improvement projects are effective, and plan new ones.
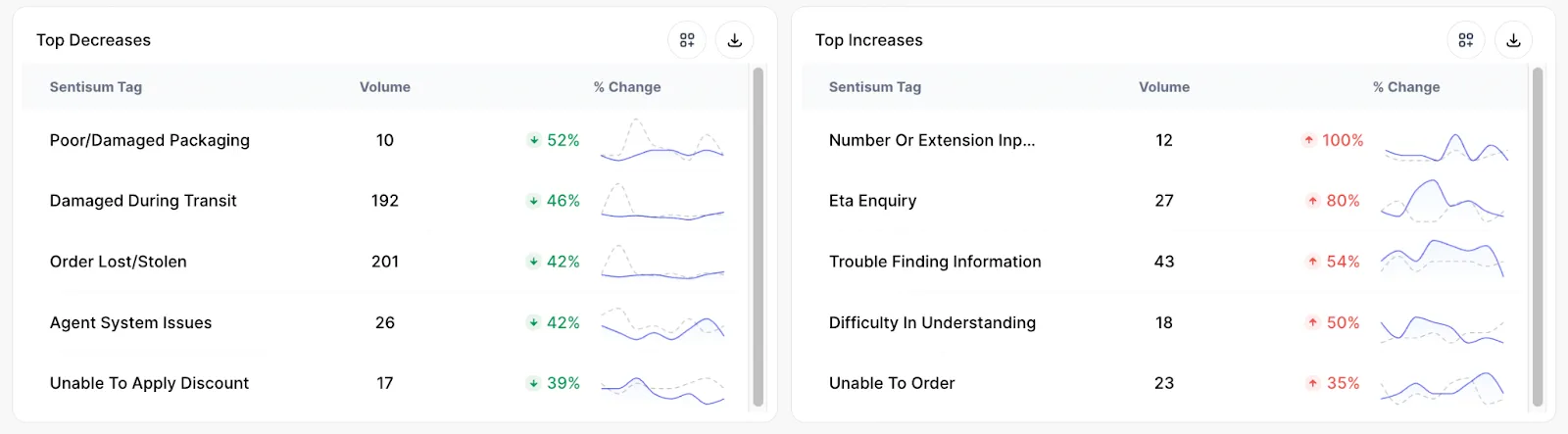
- Sentiment Analysis and Trends so you can see precisely which issues cause customer frustration and dissatisfaction.

- Top Negative and Positive Sentiment Drivers so you know which issues to prioritize fixes for
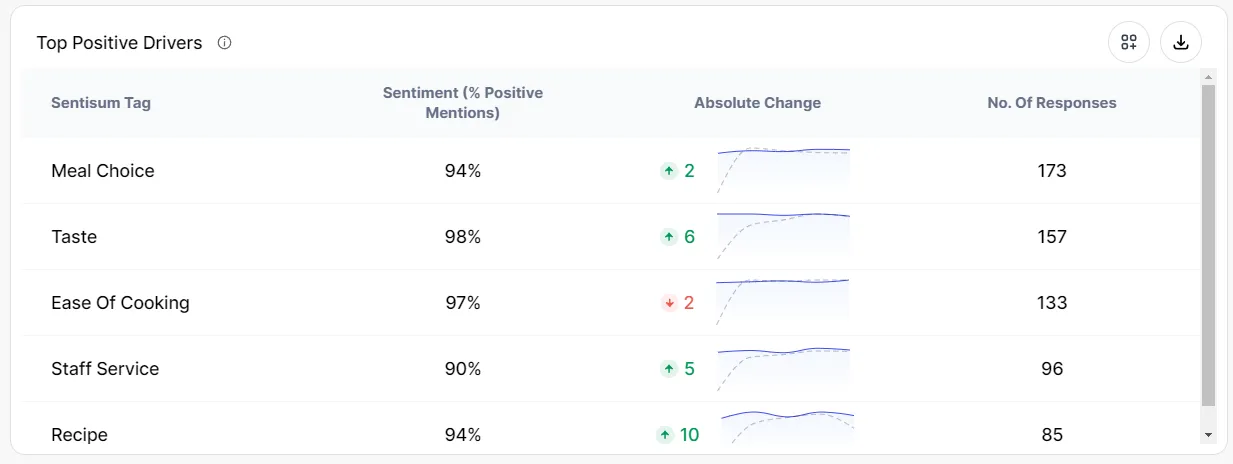

- AI Agent Performance Analysis to help you streamline quality assurance processes and agent training for better customer support.
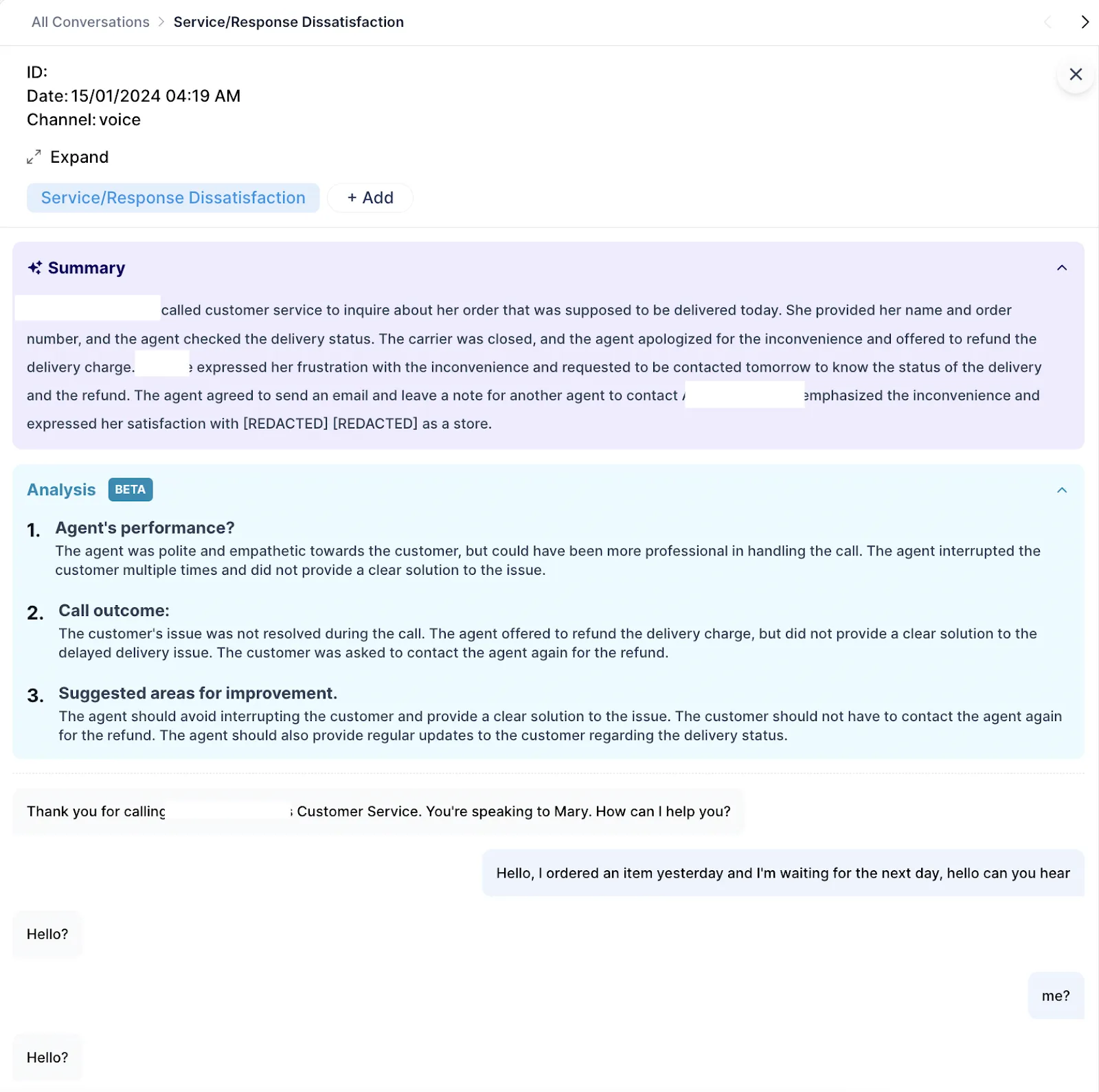
- ChatGPT-like AI called “Dig In” which allows you to ask questions about your customer data and get succinct, summarized answers.

Step 4: Insights are pushed to your help desk.
But it’s not enough to just have the insights—you want to be able to push them back to your helpdesk platforms.
On SentiSum, you can create automation workflows using a custom AI model and push triage and prioritization rules back to your helpdesk platform.
For example, you can create a rule to send a message with a highly negative sentiment and urgency level to your top-performing customer support agents so they can resolve the issue quickly and effectively.
This helps your agents understand which issues to fix first based on sentiment and urgency (e.g. angry customers need to be attended to quickly).

How Conversation Analytics Improves Customer Experience [6 Use Cases]
Customer conversation analytics is the key to happy and loyal customers.
It lets you read between the lines of your customers' emails or chats and understand what they really want.
Here are six ways customer conversation analytics can transform your customer experience and help you make better business decisions.
1. Identify Friction Points in the Customer Journey
Whenever a customer reaches out to you via an email or call, it indicates friction.
These friction points, if they blow up to bad experiences, can cause customers to churn and turn to competitors, leading to revenue loss.
As a support leader, you want to understand what these key friction areas are and how much of an impact they have on customers, so you can remove them before they turn into big issues.
With SentiSum’s automatic tagging capabilities, you can easily identify patterns and see how many people are reaching out about specific issues, without manually reading every customer conversation.
Once you know which pain points are the biggest drivers of customer complaints, you and your team can address them to reduce future friction.
💡Case Study Spotlight
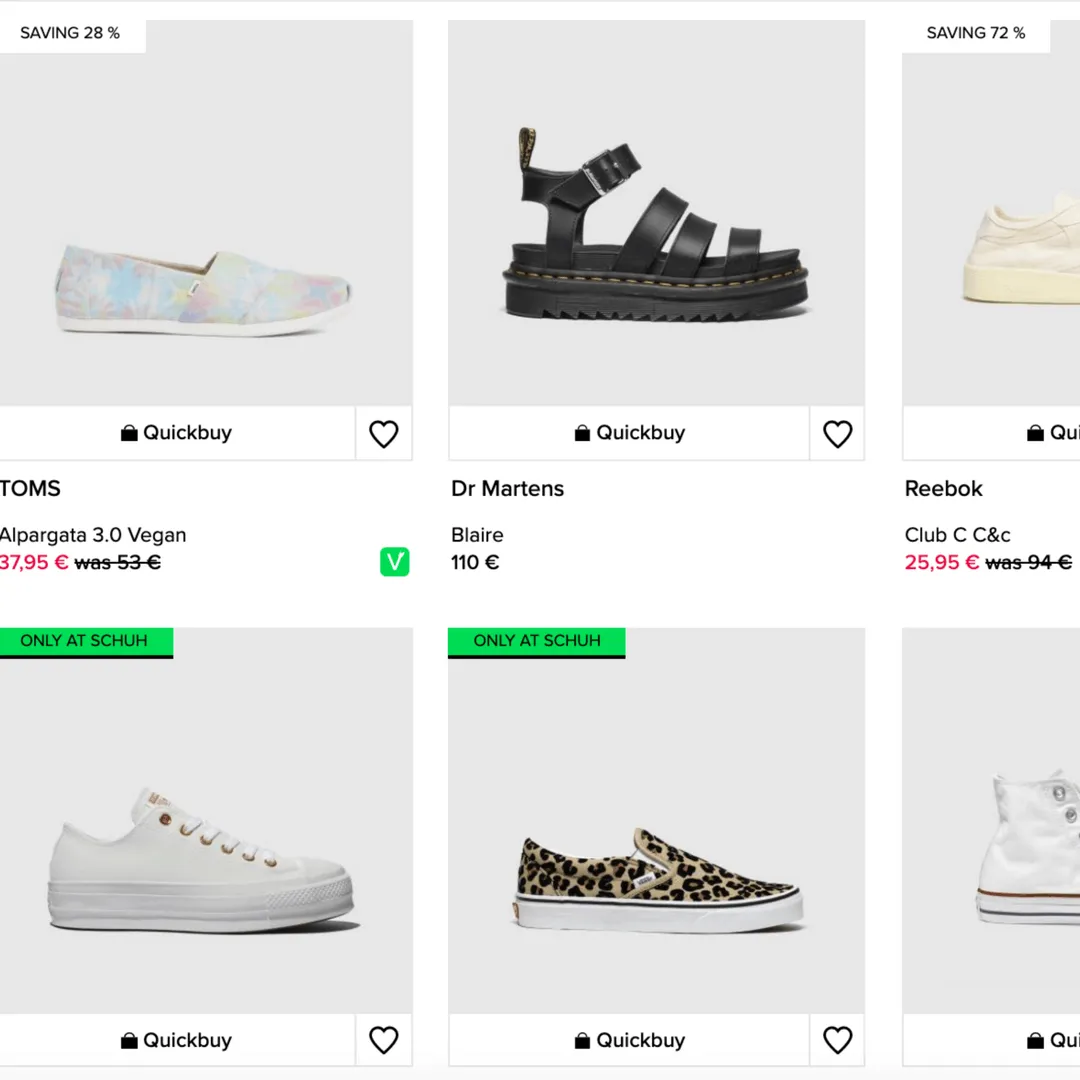
Schuh, a retailer renowned for its customer-centric approach, needed a fast way to analyze tons of customer conversations and address friction points efficiently.
With SentiSum, the company automated the analysis process and gained granular insights without manual effort.
This allowed the team to prioritize high-impact issues and improve their customers’ digital buying journey.
“Now I better understand patterns over time, which will lead the team to taking action on customer friction. Less friction means more sales."
- Sean McKee, former Director of eCommerce and Customer Experience at Schuh
2. Reduce Response Times to Critical Issues and Boost Customer Satisfaction
While it’s not always possible to solve every customer issue on the spot, a customer conversation analytics tool can help you quickly identify and address the most pressing issues first.
With SentiSum’s auto-routing and auto-prioritization functionalities, you can automatically send support conversations to the appropriate team member and implement a triage system to determine the order of urgency.
For example, a customer who’s having a checkout issue should be handled before one who’s simply inquiring about an order status, since the former will result in a new sale.
This priority system allows you to resolve critical issues extremely quickly and impress your customers.
💡Case Study Spotlight
James Villas, a villa holidays brand, took their customer support operations to the next level with SentiSum.
The team implemented an automatic triage and prioritization system to help agents respond to timely requests as quickly as possible.
The result was an impressive 46% reduction in first response time and a 51% decrease in resolution time.

“Sentisum helps us to organize our tickets and triage them to the right team member with the necessary skills to solve a ticket with the best possible outcome.”
– Johannes Ganter, Head of CRM at James Villas
3. Uncover Product Bottlenecks and Reduce Churn
Customer churn is a constant battle for many businesses.
When you analyze your conversations at scale with a customer conversation analytics tool, you can determine exactly what’s making customers walk away from your product.
This helps you capture valuable trend insights that might be missed if you only read a handful of customer conversations.
When you have broader data on the bottlenecks in your customer experience, you can fix those issues to keep people moving forward (and coming back for more).
💡Case Study Spotlight
SentiSum helped Scandinavian Biolabs transform its customer support approach by automatically tagging support tickets with relevant insights.
This objective view of customer issues and needs allowed the team to reprioritize its product roadmap.
The result? Reduced resolution times and a 50% decrease in a key driver of customer dissatisfaction.

“Support tickets … provide what you can almost view as pre-churn information. What SentiSum allows us to do is view that in an easy to understand format with a high level overview of granular information.”
– Anders Reckendorff, CEO of Scandinavian Biolabs
4. Understand Your Customers Beyond Surveys and Find Root Causes
Surveys like Net Promoter Score (NPS) and CSAT can be great for gauging customer sentiment, but they only tell part of the story.
When people fill out these surveys (if they fill them out at all), they don’t always explain why they gave the score they did.
Without an explanation of a low score, you may find yourself guessing about the real reason.
Analyzing past conversations and tying them back to the same customer’s survey score can give you a more nuanced understanding of what went wrong.
With this knowledge, you and your team can address the root cause and deliver an exceptional experience that truly impresses your customers.
💡Case Study Spotlight
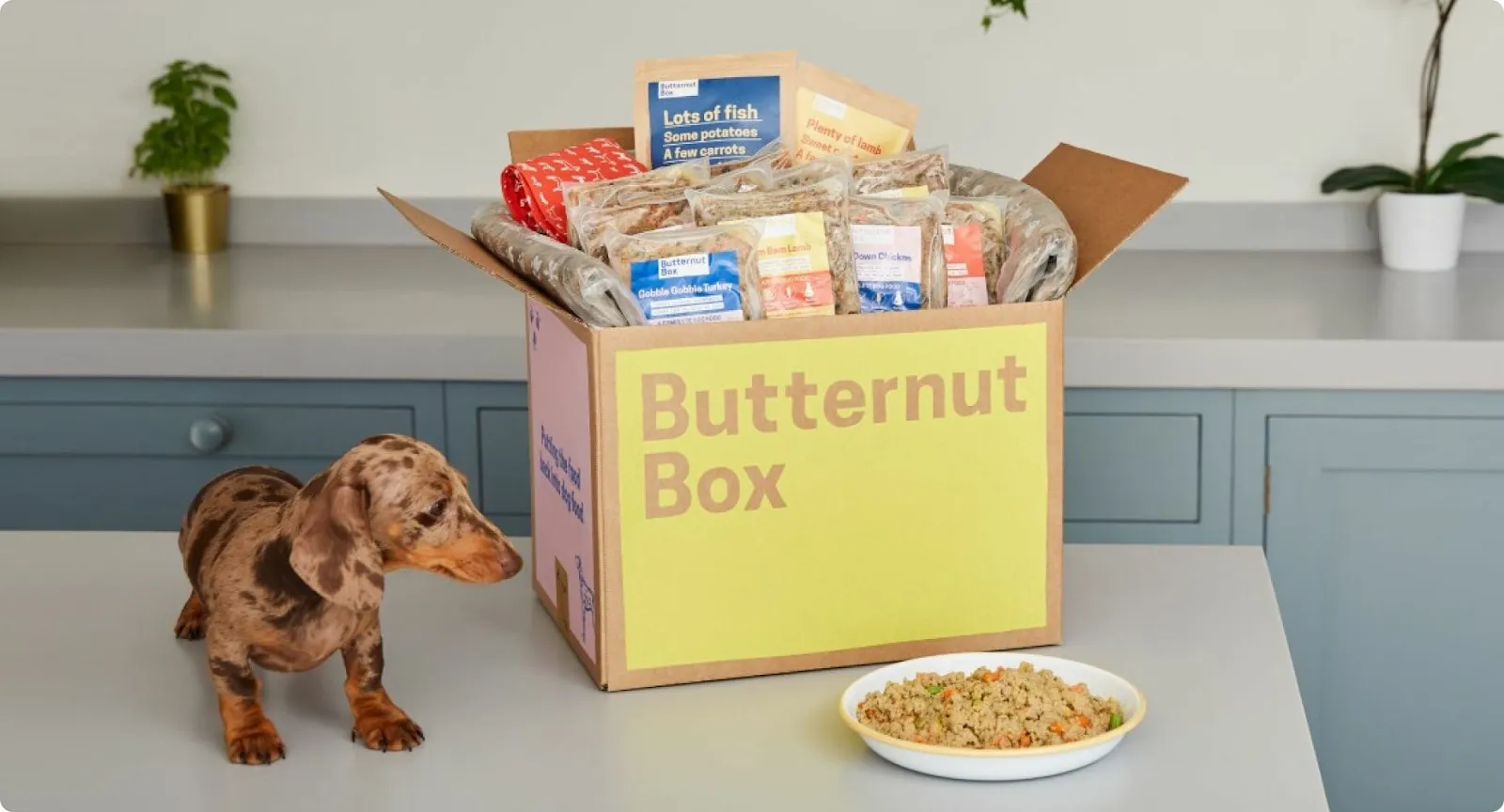
Before SentiSum, pet food subscription service Butternut Box had limited insights into the drivers behind customers’ Net Promoter Scores.
SentiSum’s automatic tagging and integration with its support platform Dixa let the team dive deeper into the root issues of low scores and focus on areas that mattered most to its customers.
Now, every department at Butternut Box can act on NPS data insights.
5. Train Customer Service Agents and Improve Service Quality
Well-trained support agents are the key to improving the customer experience.
But you can’t train them if you don’t know exactly where they’re succeeding or failing.
Reading every single customer conversation transcript across VoC channels yourself is impractical and time-consuming—but a customer conversation analytics tool can do it for you.
SentiSum allows customer support leaders to see overall trends in each agent’s performance and a summary of their customer conversations.
The software even allows you to dig into individual email threads, call transcripts, and chat logs for more context on where an agent may not have handled a customer conversation in the best way possible.
When you know your agents’ weak spots, you can focus your training efforts toward improvement and resolve specific friction points in the customer support experience.
💡Case Study Spotlight
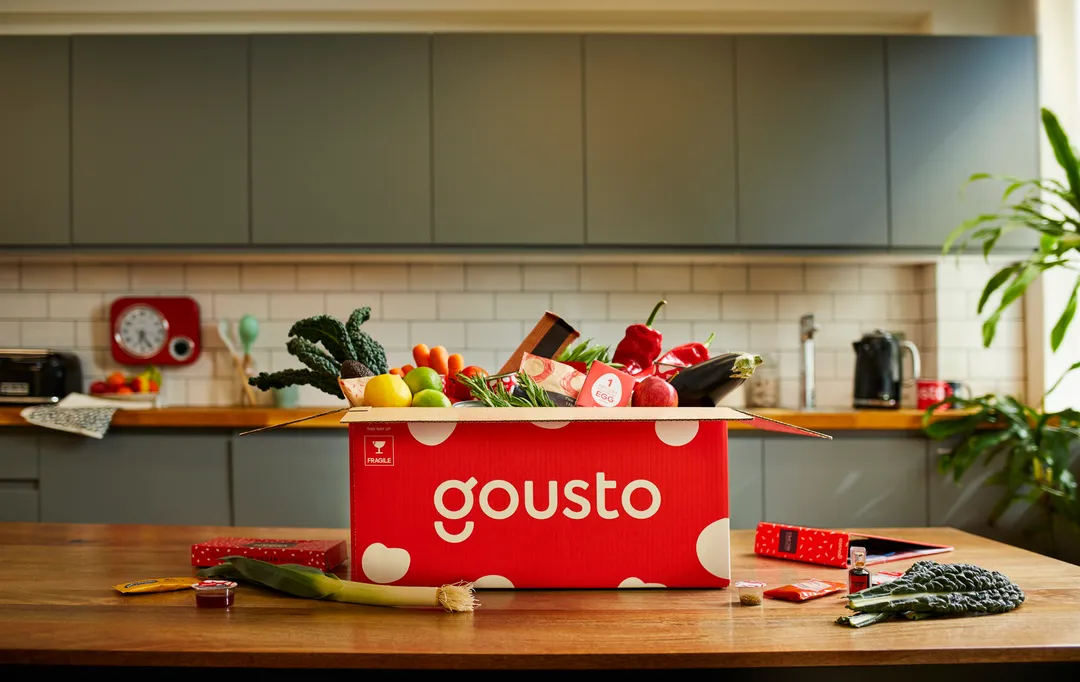
Meal kit delivery service Gousto wanted to analyze its free-text customer communication data to make comprehensive improvements to the customer experience.
By integrating all nine of its VoC channels into one platform with SentiSum, Gousto gained accurate, granular insights into customer sentiment and key topics driving contact.
This has empowered the entire company to make data-driven decisions that have reduced customer contact and improved the quality of customer service.
“By unlocking actionable insight from qualitative customer contact data, we are able to remain focused on driving overall customer experience.”
– Joe Quinlivan, Head of Customer Care at Gousto
6. Prioritize Product Fixes Based on What Customers Actually Want
The key to a successful product roadmap is knowing what your customers truly want.
Sentiment analysis gives you access to a goldmine of feedback to inform your product development plans.
By analyzing customer conversations, you can identify recurring product issues and pain points that affect your customers' experiences.
With this data, you can guide your product development efforts in the right direction.
💡Case Study Spotlight
Hotjar's Customer Success Team leveraged customer conversation analytics to integrate customer data into their product development process.
Using topic-based sentiment analysis provided by SentiSum, the team sped up the process of pinpointing specific fixes and enhancements to prioritize.
Watch our support tickets webinar with Hotjar’s Director of Support, Nick here.
“Manual processes are time consuming and bring a whole load of subjectivity. Using SentiSum, all of those [manual] steps basically disappear because it automatically categorized all of the tickets for us and displayed them in the dashboard.”
– Nick Moreton, Director of Support at Hotjar
3 Key Things to Consider When Choosing a Conversational Analytics Tool
Now that you've seen the benefits of a conversation analytics tool, you might decide to invest in one.
But not all analytics tools are created equal.
To get the best bang for your buck, we recommend you consider these three things when shortlisting tools:
#1. Choose a tool that uses machine-learning based NLP.
We highly recommend you ask the software provider these two key questions:
- What type of NLP do they use? You don’t want a simple keyword extraction NLP or rule-based NLP. To get the most accurate and granular insights, you need machine-learning based NLP that will understand text and speech like a human would.
- Can they build you a customized AI model? If they don’t plan to, it’s unlikely to provide the accuracy or granularity you need to achieve your goals.

#2. Choose a tool that anyone in the organization can easily use.
Advanced features are helpful, but they shouldn’t come at a high training cost to you.
You want everyone in your organization, whether it’s the CMO or your data analyst, to be able to use the software and access insights readily on the dashboard.
This ease of use is what really drives change across departments.
“Imagine a world where every team member, regardless of technical know-how, can grasp customer insights and use them to make informed decisions.”
- Sharad Khandelwal, CEO of SentiSum
#3. Choose a tool that integrates with your existing tech stack.
Your customer conversation likely comes with help desk platforms like Zendesk, Freshdesk, or Dixa. (SentiSum integrates with them all.)
You want a conversation analytics tool that integrates with the platforms you use, so you can not only bring data but also push automations and insights back to them.
This is crucial to set up prioritization and triage rules for your customer support team.
Book a demo with SentiSum today.
