In this article, we'll teach you how to thoroughly analyze your customer feedback (whether it comes from reviews, surveys or customer support chats).
We'll also look as some advanced feedback methodologies for readers sitting on high volumes of feedback.
Let’s dive in.
In this guide:
- What is customer feedback analysis?
- Why is analyzing feedback important?
- Customer feedback analysis methods - which should you use?
- How to analyze customer feedback manually (step-by-step with template)
- Real example of a customer feedback analysis
- Choosing the best feedback analysis tech to help out
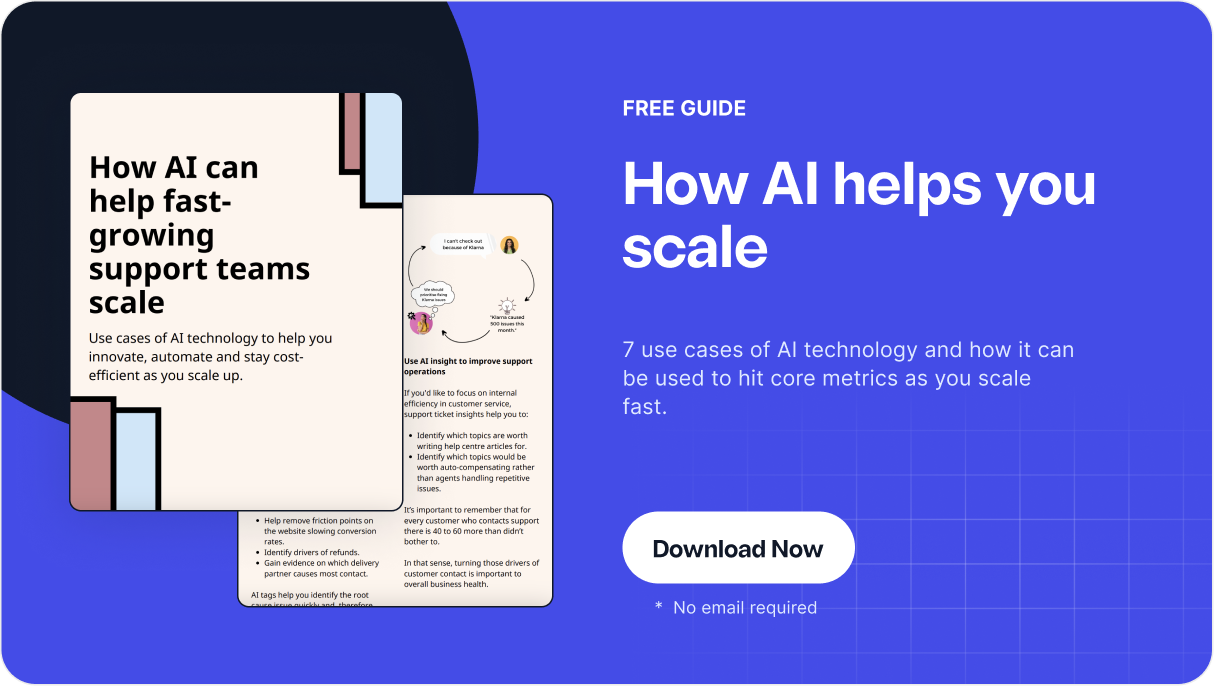
What Is Customer Feedback Analysis?
Customer feedback analysis is the process of evaluating and interpreting feedback left by your customers for your product or services.
The way you analyze feedback will depend on its source and volume—for example, if you have 100,000 support emails per month it'll be hard to analyze that feedback by hand.
As we'll cover below, cutomer support emails are just one of many ways to obtain customer feedback. Analyzing each source of feedback would likely give you different results depending on when and how it was collected.
Examples of Customer Feedback Collection Methods (And What an Analysis Would Tell You)
Most companies look to customer data sources like surveys, reviews, and customer support conversation logs to obtain customer feedback.
These sources of feedback collection each have different advantages and should be chosen based on your particular goals.
The three most reliable (and commonly used) feedback channels are CSAT surveys, Net Promoter scoring, and customer support conversation analysis.
Here's quick review of all three:
- Customer satisfaction (CSAT) surveys are typically used as a measure of transactional customer experience. This means they’re sent to customers to understand their experience on a particular touchpoint, like an interaction with the customer service team. Analysis of CSAT surveys tells you what customers like/dislike only about that particular interaction.
- Net promoter surveys (NPS) can be transactional or relational depending on how you set them up. A relationship-based NPS is a measure of customer loyalty focused on the overall, long-term relationship between a customer and your company, rather than a specific transaction or interaction. NPS specifically measures the “likelihood to refer a friend” and is used as a proxy for loyalty and brand health, so an analysis of NPS feedback gives you insight into what’s driving these.
- Customer support conversations are recorded and collected automatically by your customer service software. We recommend our clients prioritize their support conversation logs as a source of customer feedback because they’re the least biased. With surveys and reviews, who leaves them for you is skewed towards really angry or really happy customers, which means the results kinda suck. Support tickets don’t have that problem because the feedback comes passively.
Ultimately, the feedback you collect and the way you analyze it depends entirely on your goals.
Why is Analyzing Feedback Important?
Listening closely to customer feedback is widely considered critical to long-term business success. Customer feedback analysis plays an important role in helping make customer-centric decisions that improve the customer’s experiences.
Compelling research shows companies that invest in their customer’s experiences earn superior growth thanks to better customer retention and word of mouth referrals.
The business world attributes the success of companies like Amazon and Apple to how well they listen, interpret and build products around feedback. Both are well known for obsessing over their customer’s pain points and solving them better than anyone else.
One of my favourite examples of a company gaining advantages through feedback comes from the meal-kit delivery company, Gousto. The team at Gousto run a real-time analysis on all their support conversation channels to understand things like why customers are returning food or demanding a refund.
Gousto's customer frustrations, often expressed by email or chat, reveal frictions in the customer’s experience and by fixing the common reasons for returns or refunds, Gousto can both help future customers and save the company from losing money.
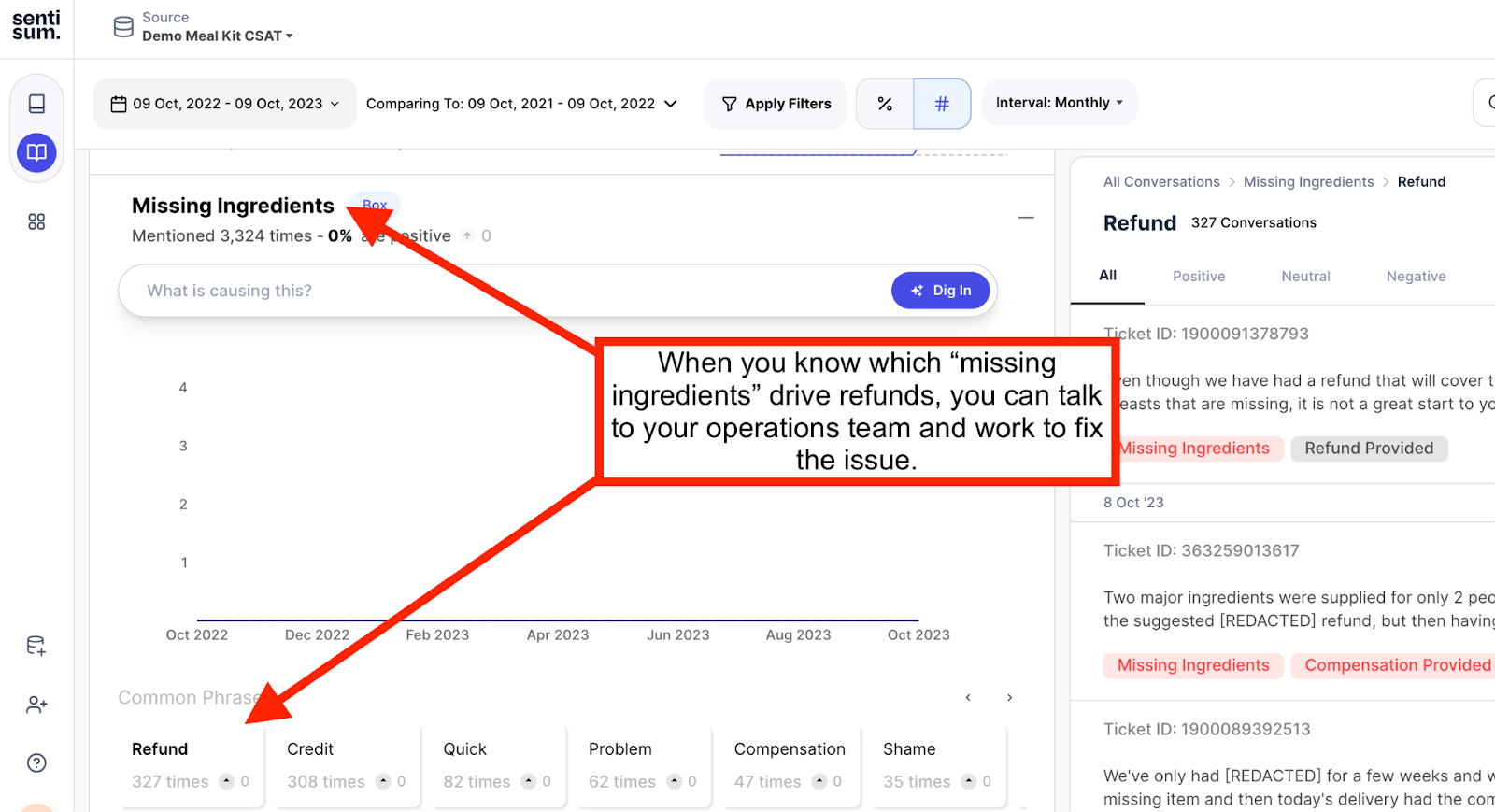
Customer Feedback Analysis Methods - Which Should You Use?
Customer feedback is generally qualitative. It’s written text left within surveys, reviews, chats, and complaints.
To effectively interpret customer feedback, it's important to transform qualitative text into quantitative data using methods like:
- Text analytics
- Sentiment analytics
These analytics tools quantify issues providing a more reliable foundation for analysis and decision-making.

If you have only 100 customer reviews and you want to analyze them, it might make sense to simply go through and categorize them by hand—a manual text and sentiment analysis (e.g. 10% mention this topic, 30% mention this topic, etc.).
For a handful of pieces of feedback, a manual analysis would be sufficient for interpreting your feedback and finding improvement points to act on—we'll teach you how to do this later on.
However, most businesses have thousands if not hundreds of thousands of qualitative survey responses, reviews and customer support interactions..
You could take a small sample, but we find that trust and credibility is an important factor in winning internal buy-in to improve and correct poor experiences.
Luckily, there’s technology that's incredbile effective at automating customer feedback analyses: AI.
In our customer feedback analysis platform, we leverage an approach to text and sentiment analytics called machine learning-based topic analysis.
The AI reads surveys, reviews, and support conversations and understands their nuance much like a human would.
It then quantifies the topics driving positive and negative sentiment, reasons for contact, and trends over time to help you understand your customer feedback.
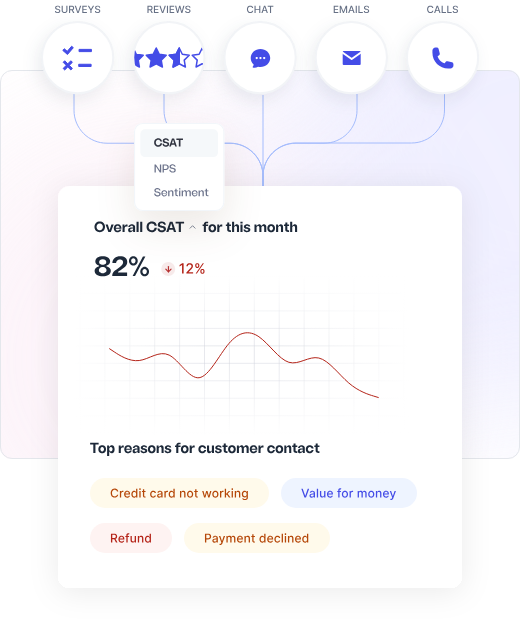
If you’re considering regularly analyzing customer feedback and have significant volumes of customer feedback, feel free to book a meeting with us here to find out more about our feedback analysis platform.
For readers who only have only a handful of pieces of customer feedback, we’re now going to show you step-by-step how to manually analyze customer feedback for topic and sentiment insights.
How to Analyze Customer Feedback Manually (Step-by-Step With Template)
Before we get stuck into the step-by-step process of feedback analytics, it’s important to note that it’s time-consuming and subjective.
This method will work best with a small number of feedback pieces, a lot of time, and just one person doing the analysis—any more may change the results because everyone interprets feedback differently.
For this manual analysis, we’re going to do a high-level ‘topic analysis’ and a general ‘sentiment analysis’ on your customer feedback.
Our goals:
- Quantify the topics your customers mention
- Understand their impact on your customers (positive, negative or neutral)
Let’s get stuck in.
Step 1: Download Our Feedback Analysis Template
We built a very simple feedback analysis template to get you started.
Click here to grab your copy now and then move to step two.
Step 2: Choose Your Feedback Channels
It’s important you know why you’re doing this feedback analysis. Once you do, choosing a feedback channel to analyze should be easy.
For example, if you’re a restaurant owner and want to understand why people are living negative Google reviews, your job is simple. Export all your Google reviews and get started.
However, if you’re looking to improve overall customer experience and want to identify the most impactful new projects to work on in 2024, it becomes more difficult.
We recommend choosing the most up-to-date and high-volume feedback dataset. If lots of customers contact your customer support each month, start there. But if you run a regular survey and don’t have customer support, take your last 6-12 months of survey data instead.
For this feedback analysis example, we choose CSAT surveys.
Step 3: Collate Your Feedback in One Place
Export your feedback and collect all the raw data in one place (e.g. an Excel sheet).
With it, include any extra information that might help later on like. For example, where did the feedback come from? Who is the customer? How much is their monthly spend with us?
You’ll want to collect the same data on each piece of feedback to have a complete dataset and a meaningful result ultimately.
Here’s the example template with columns for additional customer data:
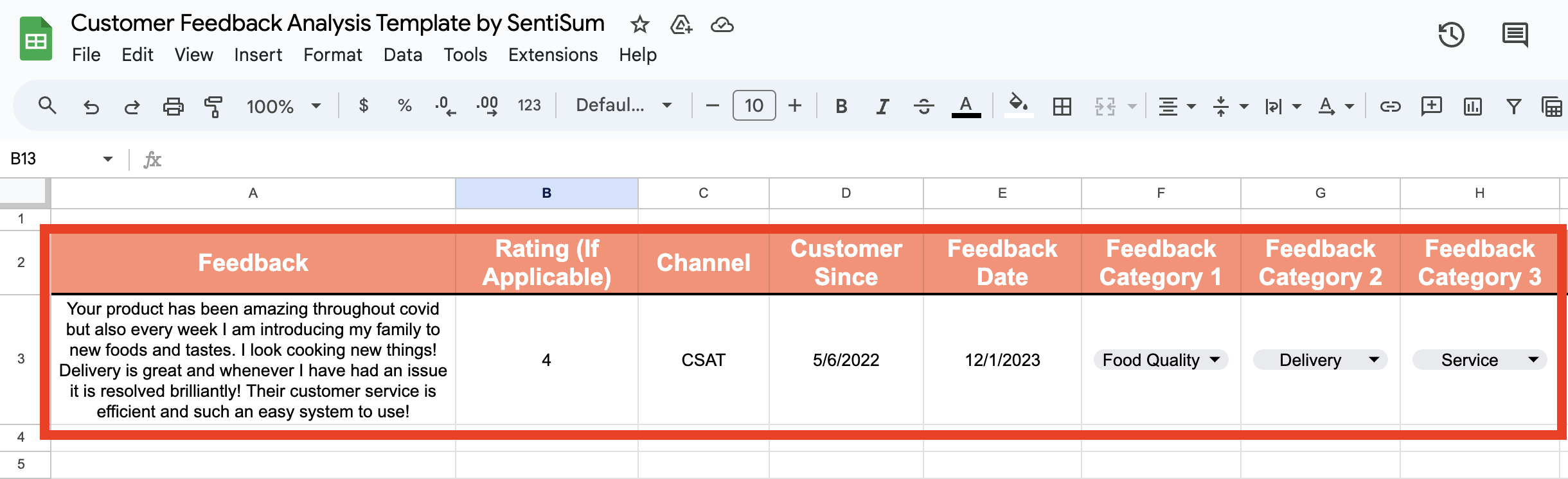
Step 4: Categorize Your Feedback
Once your customer feedback data set is in one place, you need to think about how you’re going to categorize the data.
You’ll need two spreadsheets. One for the feedback you’ve already collated, and another to store the categories, themes, and sentiments with which you’ll code the feedback.
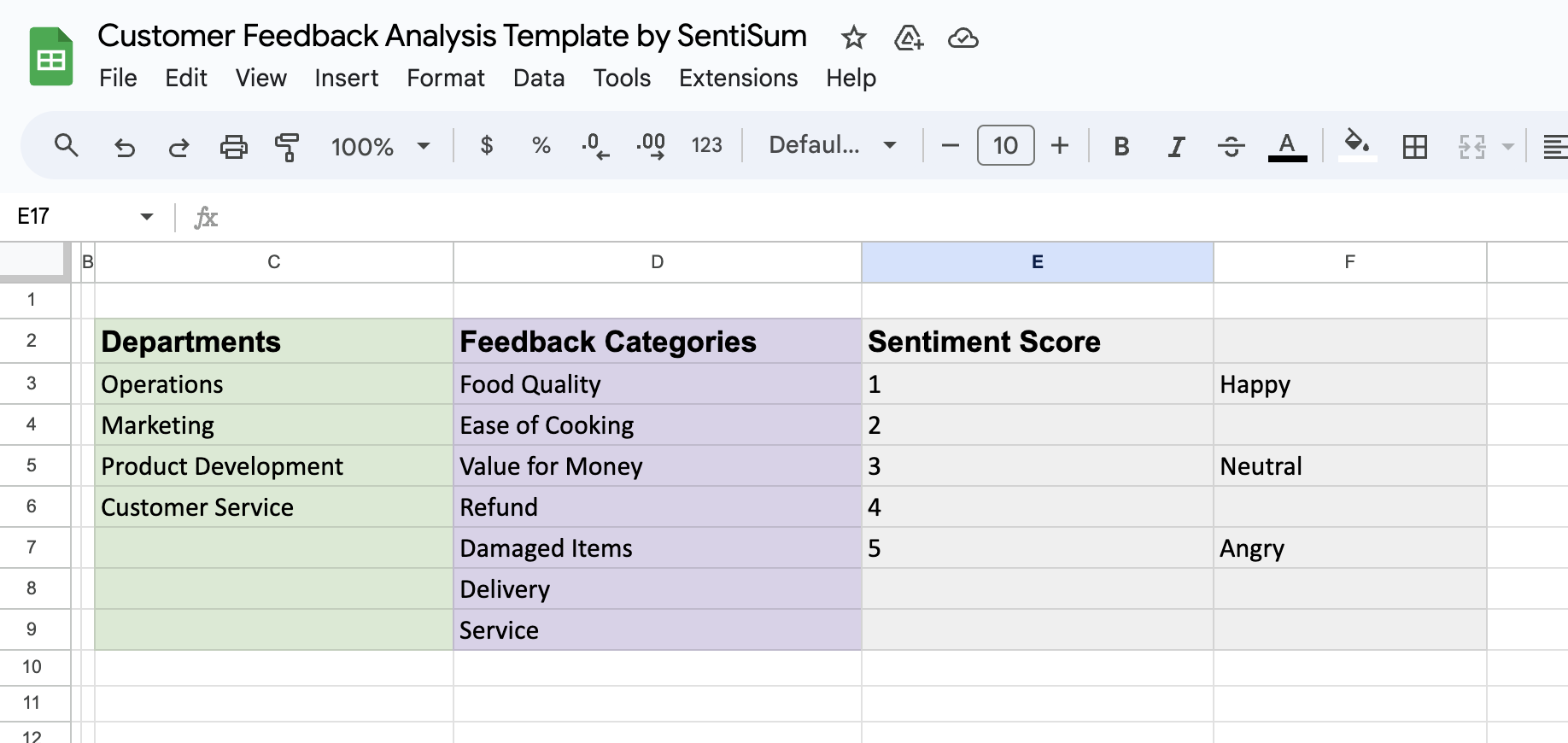
For our feedback dataset—CSAT survey results—we decided to categorize the feedback into four tags: ‘delivery issues’, ‘payment issues’, ‘refund issues’, and ‘packaging issues’.
For help choosing yours, read our guide to building a tagging taxonomy here.
We also added a two additional data columns:
- Which department will find this feedback relevant (useful for sharing it later on)
- A sentiment score (using a basic 1-5 scale)
You can expand these to as much as you like, the more detailed you can go the more complex your analysis will be but also the more actionable the results will be.
Here’s an example of our basic taxonomy applied to one survey result:
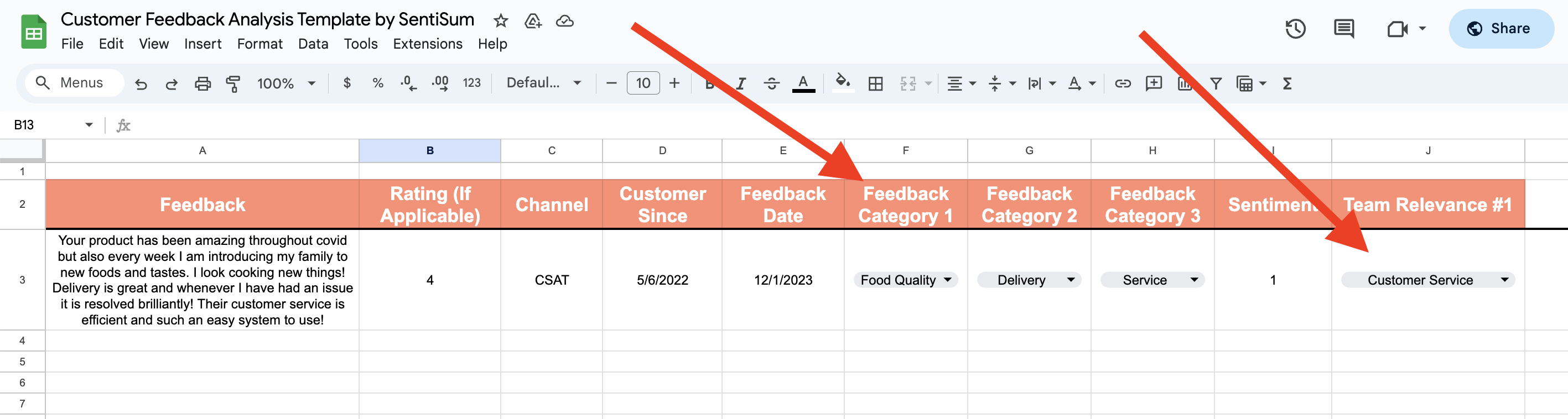
To build your own taxonomy, you should start by reaching out to colleagues across your company.
Every team has different interests and needs. If you align your customer feedback analysis with their current needs the results will be even more helpful and actionable.
Step 5: Doing a Root Cause Analysis of Customer Feedback
A root cause analysis requires you to go deep with your analysis, getting to the heart of the issue at scale.
Unlike in our example above, which uses a flat tagging structure, you’ll want to use a hierarchical tagging taxonomy.
A hierarchical taxonomy allows you to categorize feedback at multiple levels (e.g. Payment issue → Paypal isn’t working) so you can draw patterns even at a granular level.
If you’re looking to do a granular analysis, you should create a clear, codified taxonomy with which to categorize your feedback.
Step 6: Feedback Analysis Report: How to Present Customer Feedback
How you report your feedback analysis is almost as important as the analysis itself.
Your feedback analysis report needs to be understood by others and credible enough that they act upon it.
Your feedback reporting should strike a chord with the audience emotionally while filling them with confidence—both are needed to make change happen (suggested read: How to sell the value of customer experience internally).
Let’s also take a look at some examples of how you can report your feedback analysis results.
Two Feedback Report Examples
These are sample feedback reports are taken directly from our platform and should give you some ideas for presenting yours:
- Time-Series: Show Changes Over a Period of Time
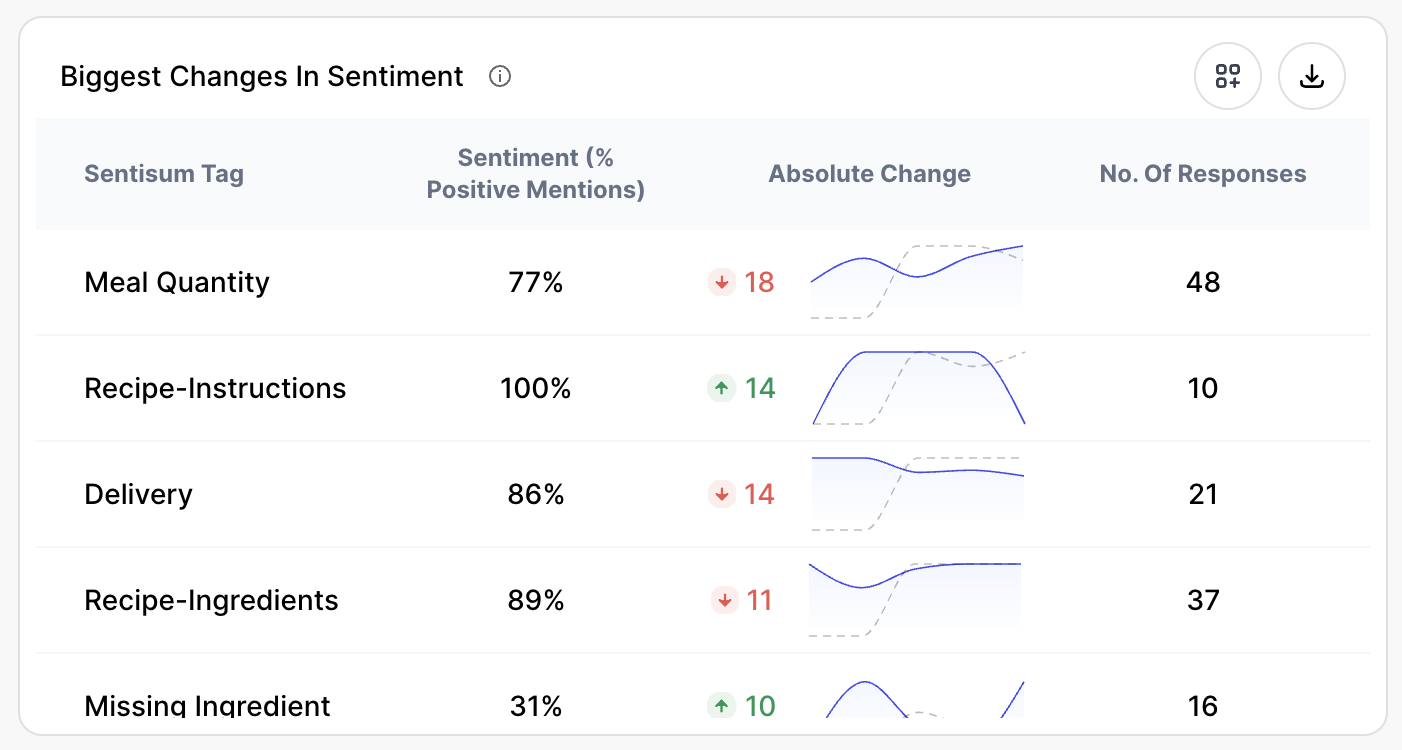
A time series report shows the change in sentiment for particular topics over time. It’s only possible if you do a regular feedback analysis and continuously update and track movements.

If you’ve recently implemented a new project, you can also drill down to a topic level to track changing the impact of your changes on customer sentiment.
Related read: Our Complete Guide to Customer Sentiment Analysis
- Topical Driver Report
This report shows you the volume of each topic, which is an indicator of the impact of an issue.

If you goal was to reduce the volume of customers contacting support, you may want to report on the topics driving them to do so. That would give your team a clear idea of what to prioritize to achieve this goal.
Top Tip: The best feedback analysis reports balance quantitative and qualitative insights. Show the hard data (500 mentions of “X”) but hit your audience in the feels with a real quote from a customer experiencing the problem.
Customer Feedback Analysis Example - Analyzing the Reviews of Revolut and Transferwise
We're lucky enough to be able to use our tool in-house to analyze unlimited volumes of customer feedback.
To show what our tool can do, back in 2021 we used our topic and sentiment analysis tool to analyze 100,000+ Trustpilot reviews for two British banking technology companies.
We compared the public Trustpilot reviews for Revolut against Wise (formely Transferwise).
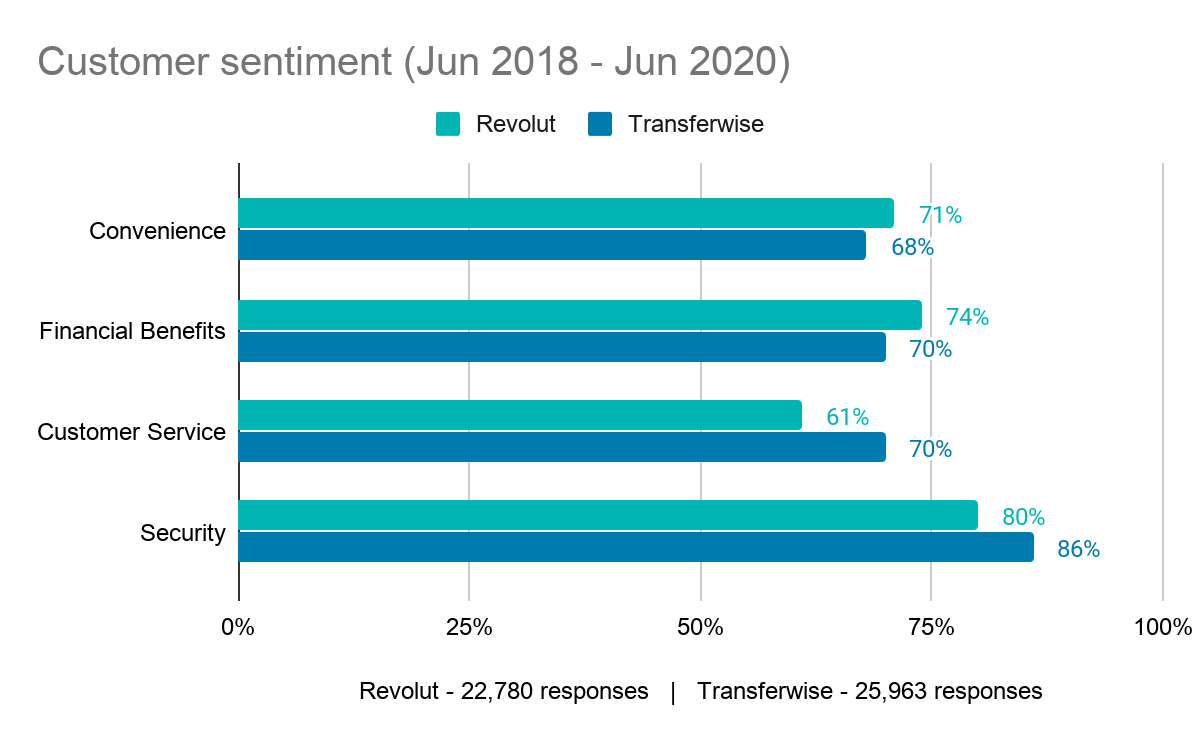
The percentage figures on the chart show how many mentions of that topic were a positive sentiment (e.g. customer service had 61% positive mentions for Revolut vs. 70% for Wise).
This analysis is high-level, focusing on only four categories that mattered most to customers. If you’d like to dive into the full feedback analysis, click here.
Choosing The Best Customer Feedback Analysis Tool For You
There is a wealth of customer feedback analysis tools to choose from, which can make it difficult to choose.
We highly recommend booking a number of product demos to look for a tool that can help you with your problem—you’ll get a good insight into the company and how they treat customers through their sales process alone.
When choosing an analytics tool, we always recommend asking these questions:
- What channels of feedback do you analyze? Some tools only do NPS, others only do reviews,very few analyze support conversations. We advise investing in a tool that covers all potential channels, to futureproof your purchasing and to ensure one platform can give you a holistic understanding of customer feedback.
- How granular and accurate are your insights? If the tool uncovers really granular insights from your customer feedback, it makes root cause analytics easier. Some automation tools do surface level analytics, which means you have to still do a lot of manual analysis to truly understand the feedback.
- What do I want to do with the analysis next? Sometimes you just need a quick analysis which you can present back to your team in a Powerpoint. Other times, you may want cross-functional teams and individuals to be able to self-serve customer insights. For the latter, you’ll want to invest in a feedback analysis tool that is easy-to-use by anyone and that includes unlimited log in’s in the package.
At SentiSum, we’ve been building customer feedback analysis software for 10+ years now. We’re trusted by large enterprises like Schuh, British Airways, and Gousto, as well as fast-growing tech companies like Hopin and Hotjar.
If you’d like to understand more about our software, please feel free to book a time slot directly with us here.